情人节过了,单身狗也想飙一把车。在这篇技术博客(资源的真义)中,日本开发者使用深度学习框架实现了根据图片检索 AV 女优的功能。
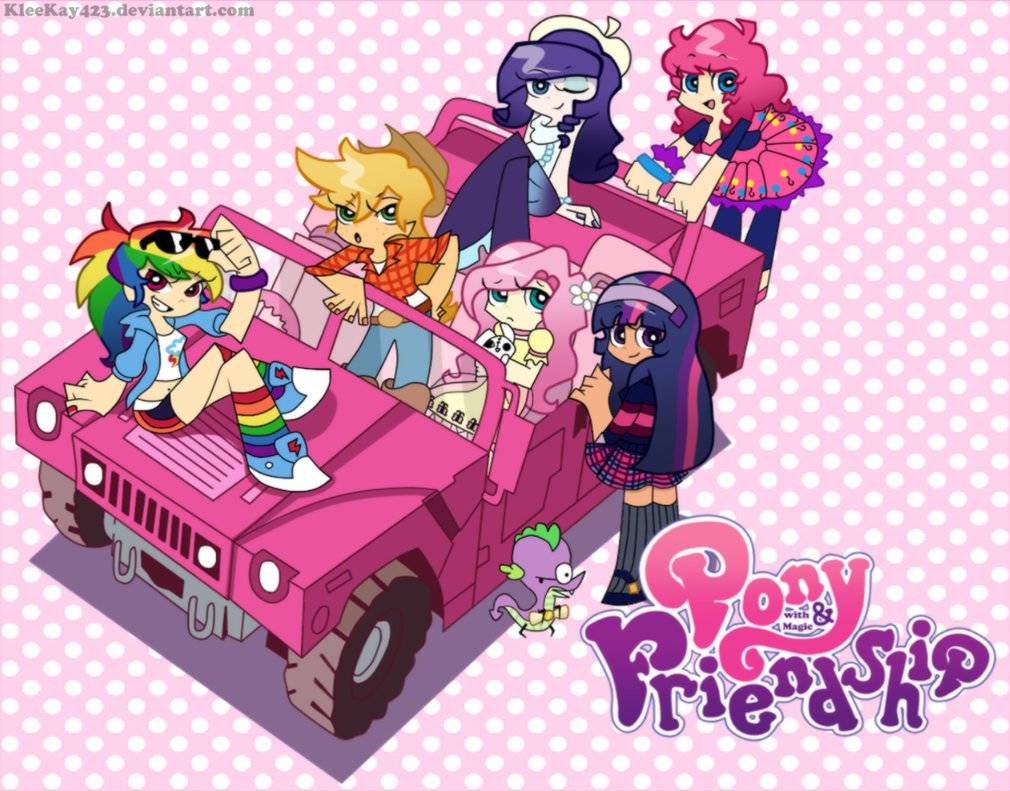
开发环境:
0. 简单的流程
(1) 收集各女优的图片
(2) 使用 dlib 提取面部图像并调整为 96*96 的大小
(3) 使用数据扩张(Data Augmentation)将女优面部图像的数据扩张到 1000 张
(4) 将数据转换为 numpy 文件
(5) 使用 Chainer 进行面部图像的学习
(6) 在完成学习后的模型下,对任意图片进行预测
1. 收集女优图片
./folder
|--- /actress1
| |--- image1.jpg
| |--- image2.jpg
| |--- image3.jpg
|
|--- /actress2
| .
| .
|--- /actress3
.
.
.
2. 使用 dlib 提取面部图像
说到图像识别,OpenCV 应该更加有名。不过在提取面部图像部分,dlib 程序库的误识别比较少,因此这里使用 dlib 可以更加精确。
使用 OpenCV 和 dlib 进行人脸识别的比较可以参考这个视频:dlib vs OpenCV face detection (https://www.youtube.com/watch?v=LsK0hzcEyHI)(译者注:youtube 的视频,有必要的话可以搬过来。)
dlib 不仅可以提取面部图像,也有识别眼睛,鼻子,脸形等要素的机能。
import os
import sys
import glob
import cv2
from PIL import Image
import dlib
"""
INPUT_DIR是收集的女优图片所在的目录名
OUTPUT_DIR是提取后的图片存放的目录名(文件夹的构成与INPUT_DIR一样)
"""
detector = dlib.get_frontal_face_detector()
# 取得各女优的目录列表
dir_list = os.listdir(INPUT_DIR)
for i, dir_name in enumerate(dir_list):
if not os.path.exists(os.path.join(OUTPUT_DIR, dir_name)):
os.mkdir(os.path.join(OUTPUT_DIR, dir_name))
image_files = glob.glob(os.path.join(INPUT_DIR, dir_name, "*.jpg"))
for j, image_file in enumerate(image_files):
img = cv2.imread(image_file)
dets = detector(img, 1)
open_img = Image.open(image_file)
for k, d in enumerate(dets):
# 丢弃尺寸小于80的图像
if d.right()-d.left() 80 or d.bottom()-d.top() 80:
continue
image_file = image_file.replace(INPUT_DIR, OUTPUT_DIR)
# 如果一张图中提取了多个人脸,则进行重命名
output_file = image_file.replace('.jpg', '_'+str(k)+'.jpg')
cropped_img = open_img.crop((d.left(), d.top(), d.right(), d.bottom()))
cropped_img.resize((96,96)).save(output_file, 'JPEG', quality=100, optimize=True)
参考资料:dlib.net face_detect.py (http://dlib.net/face_detector.py.html)
3. 数据扩张 (Data augmentation)
在深度学习的过程中,如果数据量不够大,可以人工增加训练集的大小。通过平移, 翻转, 加噪声等方法从已有数据中创造出一批"新"的数据,这就是数据扩张 (Data augmentation)。
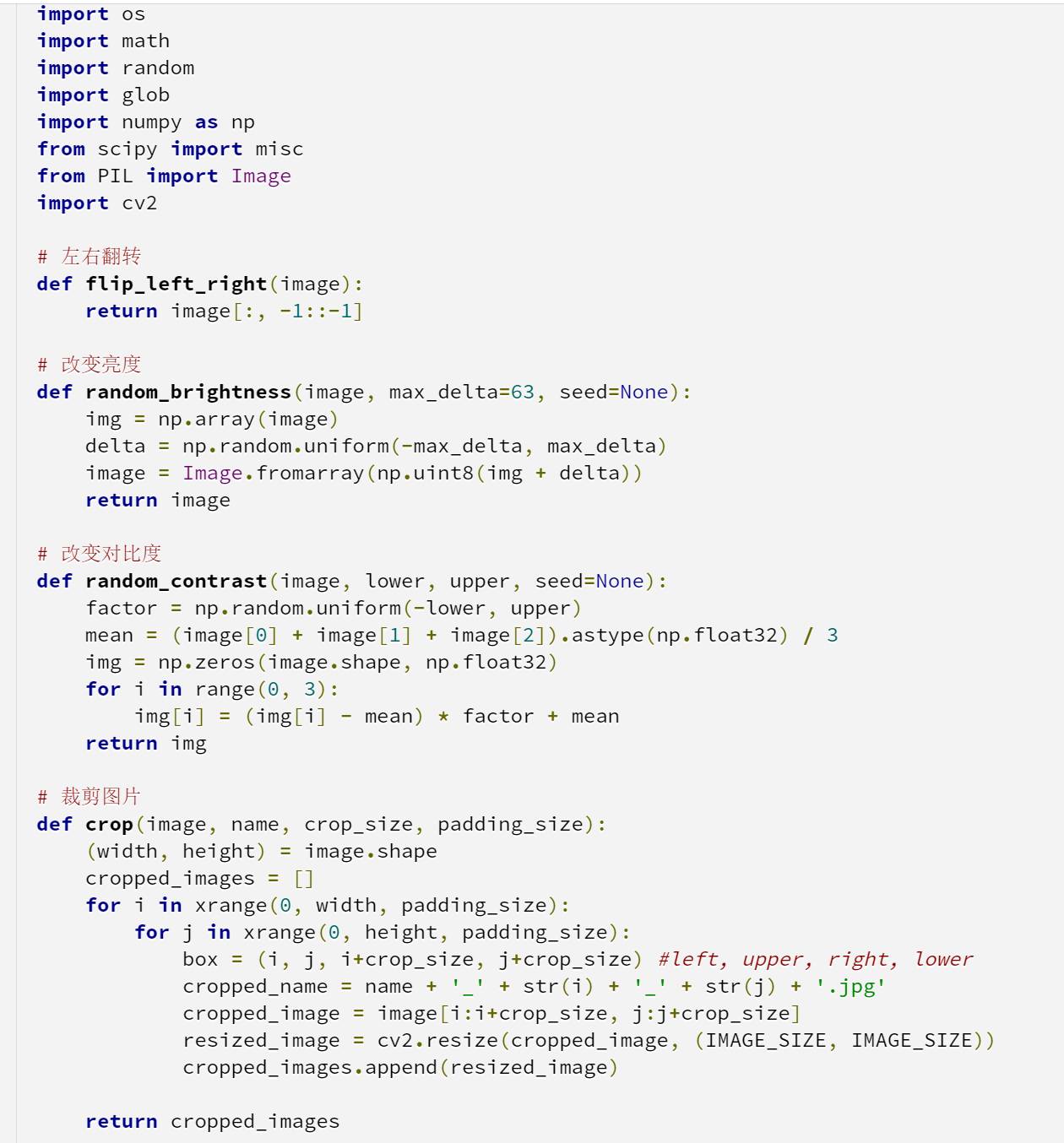
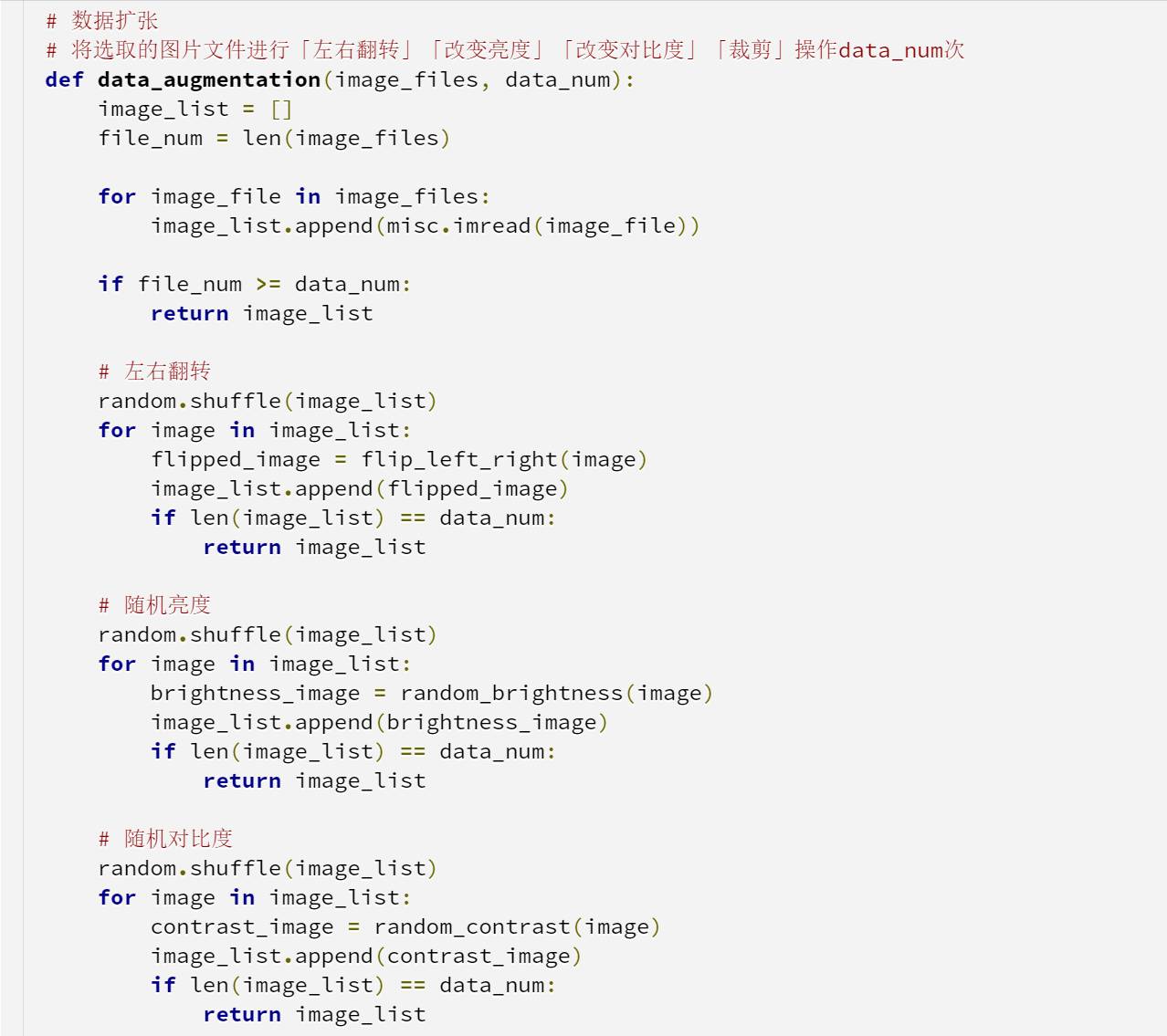
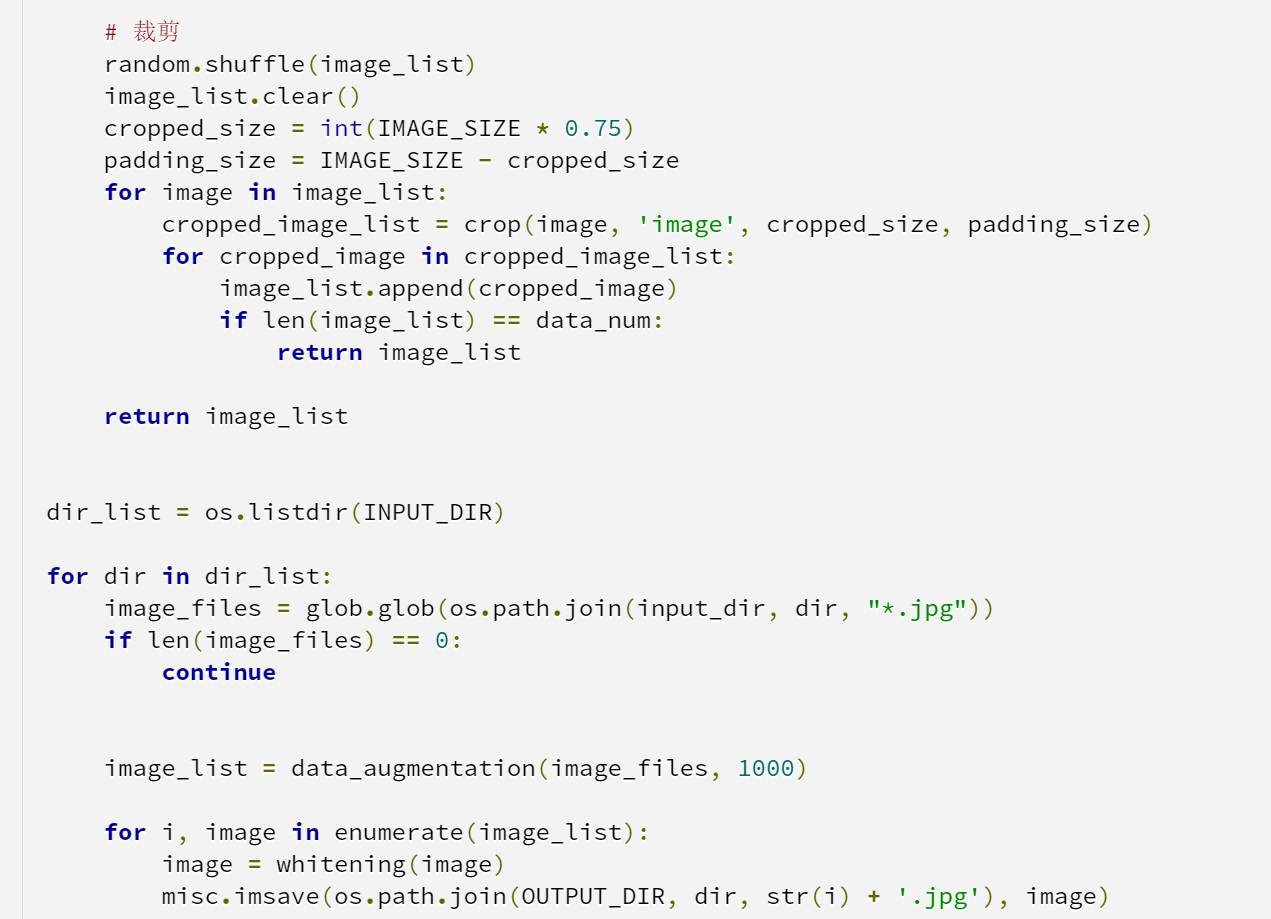
4. 将数据转换为 numpy 格式
import os
import sys
import glob
import random
import numpy as np
from scipy import misc
""" 从选择的目录里提取文件 """
def load_data_from_dir(input_dir_name, input_dir_list, start_index, test_freq):
train_list = []
test_list = []
for dir_index, dir_name in enumerate(input_dir_list):
image_files = glob.glob(os.path.join(input_dir_name, dir_name, "*.jpg"))
train_count = 0
test_count = 0
print('directory:{} index:{}'.format(dir_name, dir_index + start_index))
for file_index, file_name in enumerate(image_files):
image = misc.imread(file_name)
label = np.int32(dir_index + start_index)
if not file_index % test_freq == 0: # set train datq
train_list.append((dir_name, image, label))
train_count += 1
else:
test_list.append((dir_name, image, label))
test_count += 1
print("directory:{} total:{} train:{} test:{}".format(
dir_name, train_count + test_count, train_count, test_count))
return train_list, test_list
""" 将数据储存为numpy格式 """
def save_dataset_numpy(data_list, image_path, label_path):
image_list = []
label_list = []
for _, image, label in data_list:
image_list.append(image)
label_list.append(label)
image_data = np.array(image_list, dtype=np.float32)
label_data = np.array(label_list, dtype=np.int32)
np.save(image_path, image_data)
np.save(label_path, label_data)
for i in xrange(0, len(DIR_LIST), 10):
# 生成10个分类的文件
train_list, test_list = load_data_from_dir(INPUT_DIR, dir_list[i:i+args.interval], i, 10)
train_data_path = os.path.join(OUTPUT_DIR, 'train', 'data-{}.npy'.format(i+args.interval))
train_label_path = os.path.join(OUTPUT_DIR, 'train', 'label-{}.npy'.format(i+args.interval))
test_data_path = os.path.join(OUTPUT_DIR, 'test', 'data-{}.npy'.format(i+args.interval))
test_label_path = os.path.join(OUTPUT_DIR, 'test', 'label-{}.npy'.format(i+args.interval))
save_dataset_numpy(train_list, train_data_path, train_label_path)
save_dataset_numpy(test_list, test_data_path, test_label_path)
5. 使用 Chainer 进行面部图像的学习
一开始打算使用 Tensorflow 做,不过由于自己想实现不少额外的机能,因此改用 Chainer 进行。
最初的学习,建立了一个 Cifar-10 (http://www.cs.toronto.edu/~kriz/cifar.html)(一般物品的 10 个分类)的学习方法,来对实际收集到的数据进行学习。
失败的地方:
// 每张图片的大小
96×96×3 = 27648(byte)
// 每类图片的大小
27648×1000 = 27648000(byte) = 26.4(MB)
// 所有图片 (66类) ... 可以计算么?
26.4×66 = 1742.4(MB) = 1.7(GB)
"""
Batch iterator class
Usage:
batch_iter = BatchIter(DATA_DIR, 100)
for batch_data, batch_label in batch_iter:
batch_start_time = time.time()
x = np.asarray(batch_data, dtype=np.float32).transpose((0, 3, 1, 2))
t = np.asarray(train_batch_label, dtype=np.int32)
x = Variable(xp.asarray(x))
t = Variable(xp.asarray(t))
optimizer.update(model, x, t)
"""
class BatchIter(object):
def __init__(self, data_dir, batch_size):
self.index = 0
self.batch_size = batch_size
self.data_files = glob.glob(os.path.join(data_dir, 'data-*.npy'))
self.label_files = glob.glob(os.path.join(data_dir, 'label-*.npy'))
data_size = 0
for data in self.data_files:
loaded_data = np.load(data)
data_size += loaded_data.shape[0]
del loaded_data
self.data_size = data_size
assert len(self.data_files) == len(self.label_files), "Invalid data size."
def __iter__(self):
return self
def next(self):
if self.index >= self.data_size:
raise StopIteration()
data = np.zeros((self.batch_size, IMAGE_SIZE, IMAGE_SIZE, 3))
label = np.zeros((self.batch_size))
incremental_value = int(self.batch_size / len(self.data_files))
count = 0
for i in range(len(self.data_files)):
loaded_data = np.load(self.data_files[i])
loaded_label = np.load(self.label_files[i])
assert loaded_data.shape[0] == loaded_label.shape[0], "Loaded data size is invalid."
perm = np.random.permutation(loaded_data.shape[0])
if i + 1 == len(self.data_files): # last item
incremental_value = self.batch_size - count
idx = perm[0:incremental_value]
else:
idx = perm[0:incremental_value]
data[count:count+incremental_value] = loaded_data[idx]
label[count:count+incremental_value] = loaded_label[idx]
count += incremental_value
del loaded_data
del loaded_label
self.index += self.batch_size
return data, label
参考资料:
CNN 画像認識分野でのディープラーニングの研究動向 (http://ibisml.org/archive/ibis2013/pdfs/ibis2013-okatani.pdf)
深層畳み込みニューラルネットワークを用いた画像スケーリング (http://postd.cc/image-scaling-using-deep-convolutional-neural-networks-part1/)
CNNのチュートリアル (https://tech.d-itlab.co.jp/ml/666/)
Tensorflow
TensorFlowでアニメゆるゆりの制作会社を識別する (http://kivantium.hateblo.jp/entry/2015/11/18/233834)
TensorFlowによるディープラーニングで、アイドルの顔を識別する (http://d.hatena.ne.jp/sugyan/20160112/1452558576)
Tensor Flow: How To (http://kzky.hatenablog.com/entry/2015/12/24/Tensor_Flow%3A_How_To)
Chainer
GitHub - chainer/examples/imagenet/ (https://github.com/pfnet/chainer/tree/master/examples/imagenet)
GitHub - mitmul/chainer-cifar10 (https://github.com/mitmul/chainer-cifar10)
はじめてのアニメ顔認識 with Chainer (http://qiita.com/homulerdora/items/9a9af1481bf63470731a)
6. 使用学习后的模型对任意图像进行预测。
def
set_model
(model_name, model_path):
model_fn = os.path.basename(
'models/'
+ model_name +
'.py'
)
model = imp.load_source(model_fn.split(
'.'
)[
0
],
'models/'
+ model_name +
'.py'
).model
print(
'Load model from '
, model_path)
serializers.load_hdf5(model_path, model)
return model
def
set_optimizer
(opt_name, opt_path, model):
if opt_name ==
'MomentumSGD'
:
optimizer = optimizers.MomentumSGD(momentum=
0.9
)
elif opt_name ==
'Adam'
:
optimizer = optimizers.Adam()
elif opt_name ==
'AdaGrad'
:
optimizer = optimizers.AdaGrad()
else:
raise
ValueError
(
'Invalid architecture name'
)
optimizer.setup(model)
print(
'Load optimizer state from '
, opt_path)
serializers.load_hdf5(opt_path, optimizer)
return optimizer
def
detect_face
(image_file):
detector = dlib.get_frontal_face_detector()
#img = cv2.imread(image_file)
image = misc.imread(image_file)
dets = detector(image,
1
)
d = dets[
0
]
cropped_image = image[d.top():d.bottom(), d.left():d.right()]
resized_image = misc.imresize(cropped_image, (
96
,
96
))
return resized_image
# 载入预测模型
model = set_model(model_name, model_path)
optimizer = set_optimizer(opt_name, opt_path, model)
detected_face_image = detect_face(input_image)
# 使用载入的模型进行预测
x = np.asarray(detected_face_image, dtype=np.float32).transpose((
0
,
3
,
1
,
2
))
x = Variable(np.asarray(x), volatile=
'on'
)
pred = model.predict(x).data
# 读取label (label在创建numpy形式的文件时做成)
categories = np.loadtxt(label_path,
str
, delimiter=
"\n"
)
# 按相似分高低重新排序
score = pred.reshape((pred.size,))
result =
zip
(score, categories)
result =
sorted
(result, reverse=
True
)
results = []
for
i, (score, label) in
enumerate
(result[:
10
]):
if i ==
5
: break
print(
'num:{} score:{:.5f} label:{}'
.
format(i +
1
, score *
100
, label))
results.append({
'label'
: label,
'score'
:
str
(
round
(score *
100
,
2
))
})
7. 使用 Keras 替代 Chainer 优化学习的方式。
前面的内容,建立了一个学习模型,它可以提取一张图片上的各种要素 (如眼睛,鼻子,脸型等) 并进行分类,从而判断这张图最像哪一位女优;本节则通过计算全结合层的特征向量的相似度,来进行相似图像的检索。
比起 Chainer,Keras 更加容易使用。因此借助 Keras,最终完全实现了根据图片检索 AV 女优的这一功能。
Keras 可以使用 ImageDataGenerator 简单地进行数据的扩张。
不过,把随机的一张图像进行倾斜,变换后所得到的学习数据其实跟原图没有区别。这样的方法一般被认为会造成过度学习。
为降低输入数据冗余性,需要对数据进行 ZCA 白化。白化是降低输入数据冗余性的预处理过程,通过白化可以使得学习算法的输入具有如下性质:(i) 特征之间相关性较低;(ii) 所有特征具有相同的方差。
ZCA 白化的方法请参考以下文章:
データの白色化 - DEEPTONEWorks (http://deeptoneworks.com/2016/10/18/20161018020000/)
CIFAR-10 と ZCA whitening - まんぼう日記 (http://takatakamanbou.hatenablog.com/entry/2015/02/15/150430)
第 2 节实现了使用 dlib 进行人脸检测,本次则更进一步,把脸部图像的特征点抽出,使用仿射变换,将眼和口的位置摆正。下面是实现本功能所使用的 openface 和 facenet,两者都已经实装。
facenet/src/align_dlib.py (https://github.com/davidsandberg/facenet/blob/master/src/align_dlib.py)_ _
openface/util/align-dlib.py (https://github.com/cmusatyalab/openface/blob/master/util/align-dlib.py)
from
keras.preprocessing.image
import
ImageDataGenerator
# 读入何种data和label
data, label = load_data()
datagen = ImageDataGenerator(
zca_whitening=
True
,
rotation_range=
10
,
width_shift_range=
0.1
,
height_shift_range=
0.1
,
horizontal_flip=
True
)
datagen.fit(data)
#
将datagen.flow
代入模型的fit_generator
函数,即可扩张动态数据
model.fit_generator(datagen.flow(data, label, batch_size=
32
),
samples_per_epoch=data.shape[
0
],
nb_epoch=
100
)
现阶段的学习数据还很少,模型和超参数的调整还没有完全,暂且使用的是下面这样的模型。
def
conv_bn_relu
(x, out_ch, name):
x = Convolution2D(out_ch,
3
,
3
, border_mode=
'same'
, name=name)(x)
x = BatchNormalization(name=
'{}_bn'
.
format
(name))(x)
x = Activation(
'relu'
, name=
'{}_relu'
.
format
(name))(x)
return
x
def
face_model
(input_shape=(
3
,
224
,
224
), nb_classes, weights_path=
None
):
inputs = Input(shape=input_shape, name=
'input'
)
x = conv_bn_relu(inputs,
64
, name=
'block1_conv1'
)
x = conv_bn_relu(x,
64
, name=
'block1_conv2'
)
x = MaxPooling2D((
2
,
2
), strides=(
2
,
2
))(x)
x = conv_bn_relu(x,
128
, name=
'block2_conv1'
)
x = conv_bn_relu(x,
128
, name=
'block2_conv2'
)
x = MaxPooling2D((
2
,
2
), strides=(
2
,
2
))(x)
x = conv_bn_relu(x,
256
, name=
'block3_conv1'
)
x = conv_bn_relu(x,
256
, name=
'block3_conv2'
)
x = conv_bn_relu(x,
256
, name=
'block3_conv3'
)
x = MaxPooling2D((
2
,
2
), strides=(
2
,
2
))(x)
x = conv_bn_relu(x,
512
, name=
'block4_conv1'
)
x = conv_bn_relu(x,
512
, name=
'block4_conv2'
)
x = conv_bn_relu(x,
512
, name=
'block4_conv3'
)
x = MaxPooling2D((
2
,
2
), strides=(
2
,
2
))(x)
x = Flatten()(x)
x = Dense(
4096
, activation=
'relu'
, name=
'fc1'
)(x)
x = Dense(nb_classes, activation=
'softmax'
, name=
'predictions'
)(x)
model = Model(
input
=inputs, output=x)
本次的模型,是将最终层之前一层的全结合层特征向量提取出来,与各女优面部图像提取的特征向量计算余弦值,来判断与各女优的相似程度。
import
numpy
as
np
from
scipy.spatial.distance
import
cosine
from
keras.models
import
Model, model_from_json
from
keras.preprocessing
import
image
from
keras.preprocessing.image
import
img_to_array
def
calculate_similarity
():
#
载入模型
model_json =
open
(
'face_model.json'
).read()
base_model = model_from_json(model_json)
base_model.load_weights(
'face_model.h5'
)
#
建立提取全结合层和4096
维特征向量的模型
model = Model(
input
=base_model.
input
, output=base_model.get_layer(
'fc1'
).output)
image_file =
'image.jpg'
img = image.load_img(image_file, target_size=(
96
,
96
))
x = img_to_array(img)
x = np.expand_dims(x, axis=
0
)
features = model.predict(x)
features = features.flatten().tolist()
# actress_features
是之前计算好的各女优面部的特征向量
#
计算输入图片所提取的特征向量和各女优特征向量余弦值的相似度
score =
1
- cosine(features, actress_features)
8. 建立服务器
这部分跟深度学习完全没有关系。一开始尝试使用了 Heroku 来建立网站,不过最终使用的是 Conoha。
dlib 和 chainer 在 Heroku 的服务器上安装非常困难。Conoha 的运行效率虽然有点问题,不过倒是很好的完成了 dlib 和 chainer 的安装。
樱花 VPS 也是一个不错的选择,不过樱花服务器没有初次使用的优惠,而 Conoha 则有针对新用户的免费方案。
原文来源:
1.chainerによるディープラーニングでAV 女優の類似画像検索サービスをつくったノウハウを公開する
2.KerasでAV 女優の類似画像検索機能を実装する
最后
想直接要作者的实现?
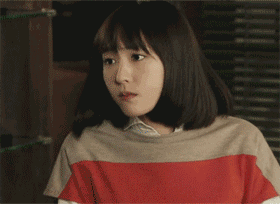
©本文为机器之心编译,转载请联系本公众号获得授权。
✄------------------------------------------------
加入机器之心(全职记者/实习生):[email protected]
投稿或寻求报道:[email protected]
广告&商务合作:[email protected]