1. Journal of Universal Computer Science
-----------------------
全文截稿:2017-10-15
期刊:Journal of Universal Computer Science
专刊:Special Issue on Advances in Security and Privacy of Multimodal Interfaces
领域:计算机科学理论
难度:★★
影响因子:0.546
网址:http://www.jucs.org/jucs
-----------------------
With the rapid development and increasing complexity of multimodal interfaces using multiple modalities of communication in human-computer systems such as speech, tactile, gestures, gaze, head and body movements, facial expressions, gait, electroencephalogram (EEG) and electromyogram (EMG) signals, the user requirements for trust, security, and privacy are becoming more and more demanding.
Multimodal interfaces developed for man-machine interactions bring together different computing paradigms such as Physiological Computing, Affective Computing, and Sentimental Computing. Security and privacy are important parts of these systems, and pose open and challenging problems. As the measures employed by the traditional security technologies may not be applicable in every case, we need to build improved multimodal interfaces for various applications in Ambient Assisted Living (AAL), Enhanced Living Environments (ELE), Assistive Technologies, Smart Houses, Internet of Things (IoT) and similar environments that will allow users to enjoy more multimodality of interactions while preserving trust, security, and privacy without losing performance, usability, and affordability of man-machine interactions at the same time.
The main objective of this special issue is to collect contributions by leading-edge researchers from academia and industry, and show the latest research results in the rapidly developed field of security and privacy in multimodal interfaces, therefore providing a valuable information venue to researchers as well as practitioners. Manuscripts regarding novel algorithms, architectures, implementations, and experiences are welcome.
2. Multimedia Tools and Applications
-----------------------
全文截稿:2017-10-15
期刊:Multimedia Tools and Applications
专刊:Special Issue on Content Based Multimedia Indexing
领域:计算机图形学与多媒体
难度:★★★
CCF分类:C类
影响因子:1.331
网址:http://www.springer.com/journal/11042/about
-----------------------
Multimedia indexing systems aim at providing user-friendly, fast and accurate access to large multimedia repositories. Various tools and techniques from different fields such as data indexing, machine learning, pattern recognition, and human computer interaction have contributed to the success of multimedia systems. In spite of significant progress in the field, content-based multimedia indexing systems still show limits in accuracy, generality and scalability. The goal of this special issue is to bring forward recent advancements in content-based multimedia indexing. In addition to multimedia and social media search and retrieval, we wish to highlight related and equally important issues that build on content-based indexing, such as multimedia content management, user interaction and visualization, media analytics, etc. The special issue will also feature contributions on application domains, e.g., deep learning for multimedia indexing, sparse data learning, cultural heritage and synergetic media production.
-----------------------
全文截稿:2017-11-15
期刊:Neurocomputing
专刊:Special Issue on Chinese Conference on Computer Vision 2017
领域:人工智能
难度:★★★
CCF分类:C类
影响因子:2.392
网址:http://www.journals.elsevier.com/neurocomputing/
-----------------------
Chinese Conference on Computer Vision (CCCV) is organized by China Computer Federation (CCF), jointly organized by CCF Professional Committee of Computer Vision. CCCV is held every two years, and is the toppest-level academic activity in the Chinese computer vision field. It has attached extensive attendance of Chinese computer vision researchers in both academic and industrial circles, and has greatly prompted the exchanges and cooperation between them.
CCCV 2017 (http://ccf-cccv.org/2017/en/) will be held in Tianjin, China on Oct. 12-14, 2017. This year's conference will consider a wide range of advanced topics related to computer vision, and also include several workshops and tutorials organized and reported by the high-level research groups in China. Multiple companies, including Nvidia, SenseTime, Megvii, HISCENE, etc. will also donate funds to support the conference and many of those also exhibit at the conference. We believe this conference will attract research papers on most advanced research topics with high-quality and innovative ideas from both academia and industry.
The main interest of this special issue, as well as CCCV 2017, includes new advances in computer vision. We are soliciting original contributions of researchers and practitioners from academia as well as industry, which address a wide range of theoretical and application issues. The Survey/Vision/Review papers are welcome.
4. Journal of Systems and Software-----------------------
全文截稿:2017-12-22
期刊:Journal of Systems and Software
专刊:Special Issue on Software Engineering Education and Training
领域:软件工程/系统软件/程序设计语言
难度:★★★★
CCF分类:B类
影响因子:1.424
网址:http://www.journals.elsevier.com/journal-of-systems-and-software/
-----------------------
The Conference on Software Engineering Education and Training (CSEE&T) is now approaching 30 years of longevity. Software engineering education has changed a great deal in that timeframe. In 1986, software engineering education was largely undertaken by industry, with just a few academic software engineering programs in place. The Master of Software Engineering (MSE) reference curriculum changed the landscape of software engineering, resulting in many MSE programs worldwide, not to mention software engineering tracks within Computer Science (CS) masters' programs. In the years that followed, software engineering education emerged at the undergraduate level, with a documented reference curriculum. Software engineering professionalism initiatives resulted in the certification and licensing of software engineers in a number of countries worldwide. We are seeing increased attention to software engineering specialty areas, and many software engineering degree programs have tracks to support these specialties.
On the industry side we are seeing an upheaval in software engineering as we know it. Software engineering is pervasive. Innovations such as Cloud Computing, autonomous vehicles, drones, bioengineering, and other initiatives have made for a rapidly changing landscape. Topics such as software assurance, safety, and reliability have become increasingly important knowledge areas.
As educators we are challenged to keep up with the emerging trends, to identify suitable software engineering techniques, and to incorporate them into our class offerings. We are in a global economy with a software supply chain that can extend across many countries and regions. Each one has their own regulations and laws about safety, security, and privacy. Practicing software engineers change jobs frequently, so the value of in-house training is not as clear as it once was.
In 2017 the conference will to focus on the changes that are taking place in the field, our response to it, and our vision of how we can move forward to meet the ever-increasing demand for qualified software engineers. Furthermore, the 2017 conference has teamed up with the Journal of Systems and Software (JSS) to devote a special issue of the JSS to the select papers from this year's conference.
Authors interested to contribute to the JSS special issue should consider submitting their preliminary work to the CSEE&T.
5. Pattern Recognition Letters-----------------------
全文截稿:2018-01-31
期刊:Pattern Recognition Letters
专刊:Special Issue on Learning Compact Representations for Scalable Visual Recognition and Retrieval
领域:人工智能
难度:★★★
CCF分类:C类
影响因子:1.586
网址:http://www.journals.elsevier.com/pattern-recognition-letters/
-----------------------
With the explosive growth of visual data, traditional hand-crafted features or learning-based representations will induce inapplicable computational complexity and large memory costs, due to exhausting computations in large-scale and high-dimensional feature space. Therefore, these conventional methods are lack of scalability for large-scale visual applications, e.g. image/video recognition and retrieval. It is highly desirable to learn much more compact feature representations to reduce computational loads for massive visual data and make big data analysis more feasible. In recent years, compact feature learning algorithms have been intensively exploited and attracted broad interests in visual analysis. For instance, benefiting from the hashing technique, we can obtain compact binary representations, based on which efficient XOR computations in the Hamming space can operate in constant time. The above compact feature learning approaches have been proved to achieve promising performance in various large-scale visual applications, such as content-based image/video retrieval and recognition. In addition, these techniques will be essential for the applications on portable/wearable devices.
The special issue will focus on the most recent progress on compact representation learning for a variety of large-scale visual data analysis, such as content-based image/video retrieval, image/video recognition, annotation and segmentation, object detection and recognition, visual processing and affective computing. This special issue will also target on related feature selection, subspace learning and deep learning techniques, which can well handle large-scale visual tasks. The primary objective of this special issue fosters focused attention on the latest research progress in this interesting area.
The special issue seeks for original contributions of work, which addresses the challenges from the compact representation learning and the related efficient representation learning algorithms for large-scale visual data.
6. Pattern Recognition Letters
-----------------------
全文截稿:2018-03-31
期刊:Pattern Recognition Letters
专刊:Special Issue on Multiple-task Learning for Big Data (ML4BD)
领域:人工智能
难度:★★★
CCF分类:C类
影响因子:1.586
网址:http://www.journals.elsevier.com/pattern-recognition-letters/
-----------------------
Big Data is a term that describes the large volume of data-both structured and unstructured. With the rapid development of new information technologies such as smart phone, mobile game platforms, smart home devices, smart health devices, and wearable computation devices, the amount of created and stored data on global level is almost inconceivable and it just keeps growing. These data is s- large and complex that traditional data processing applications are incapable of dealing with them. There are many challenges when addressing big data problem, such as data acquisition, data curation, data storage, data search, data transfer and sharing, data visualization, data query and retrieval, information security, and data analysis (e.g., prediction, user behavior analysis). Big data requires novel data processing techniques t- solve some of these challenges jointly, which is related t- Multiple-task Learning methodologies.
Multiple-task Learning is a subfield of machine learning in which multiple learning tasks are solved at the same time, while exploiting commonalities and differences across tasks. This can result in improved learning efficiency and prediction accuracy for each task-specific model, when compared t- training each of the models separately. Multiple-task Learning is an approach of inductive transfer that improves generalization by using domain information contained in the training signals of related tasks as inductive bias. It does this by learning multiple tasks in parallel using a shared representation, based on the assumption that all tasks can help each other in learning. MTL works because regularization induced by requiring an algorithm t- perform well on a related task can be superior t- regularization that prevents overfitting by penalizing all complexity uniformly. MTL may be particularly helpful if all related tasks share significant commonalities and are slightly under sampled. With big structured and unstructured data, different tasks on the same data or related data are suitable for MTL framework. Therefore, Multiple-task Learning for Big Data (MTL4BD) have broad applications in many fields, such as online recommendation system, smart home, smart health care, robotics, medical imaging, multimedia application, computer vision, human computer interaction and language processing, etc. We plan t- receive about 45 paper submissions from around 30 Universities and research institutions and we will accept about 15 papers plus a review paper totally.
7. Pattern Recognition Letters
-----------------------
全文截稿:2018-06-30
期刊:Pattern Recognition Letters
专刊:Special Issue on Learning and Recognition for Assistive Computer Vision
领域:人工智能
难度:★★★
CCF分类:C类
影响因子:1.586
网址:http://www.journals.elsevier.com/pattern-recognition-letters/
-----------------------
Assistive Computer Vision refers to systems that support people with physical and mental problems to better perform daily tasks enhancing their quality of life. The advances in learning and recognizing patterns are allowing a point of view in the definition and development of more efficient and effective assistive frameworks.
In the light of this, it is important to collect the most recent advancements in learning and recognition algorithms to be exploited in different applications to be employed to assist the modern society.
The aim of the special issue is to gather papers in which machine learning and pattern recognition are the key core in the design of advanced assistive computer vision systems to help human in tasks such as:
- Rehabilitation
- Training
- Mobility
- Assessment and diagnosis of physical and cognitive diseases
- Improving quality of Life
- Remote Healthcare
- Safe and security
- Remote Surgery
- Ambient Assisted Living
- Augmented Perception, Attention and Memory
We will invite authors to contribute with high quality paper that will stimulate the research community on building theory and applications of machine learning and pattern recognition to be used in real-life environments for assistive computer vision technologies.

Call4Papers App上线啦!下载Call4Papers App,获取更多详细内容!欢迎各位用户多提宝贵建议!
注意:请点开图片后,提取二维码,进入下载页面。
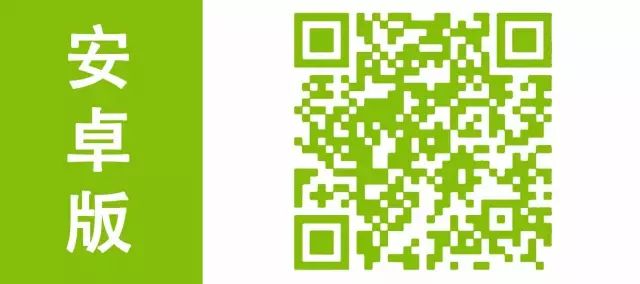
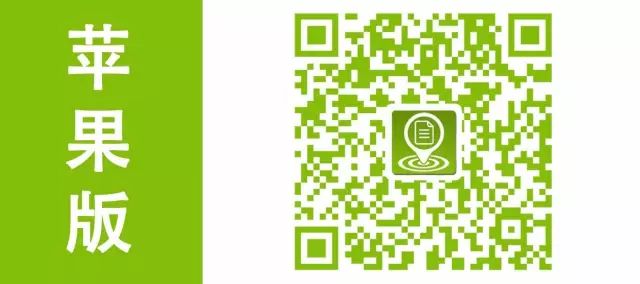