介绍一篇收录在《IEEE TRANSACTIONS ON INFORMATION THEORY》的论文。
在上一篇《结构熵理论及其应用(二)》中我们详细讲述了高维结构信息、编码树、最小结构熵等核心定理。在此基础上,本文我们将重点讲述近期中多篇顶会对结构熵的应用分类与对应文章。
Table of Contents
一、无监督/半监督聚类/社区检测
1.《SEC:More Accurate Clustering Algorithm via Structural Entropy》
2.《Semi-Supervised Clustering via Structural Entropy with Different Constraint》
二、社交事件/社交机器人检测
1.《Hierarchical and Incremental Structural Entropy Minimization for Unsupervised Social Event Detection》
2.《Adversarial Social bots Modeling Based on Structural Information Principles》
3.《SeBot: Structural Entropy Guided Multi-View Contrastive Learning for Social Bot Detection》
4.《Unsupervised Social Bot Detection via Structural Information Theory》
三、角色识别
1.《Effective and Stable Role-Based Multi-Agent Collaboration by Structural Information Principles》
一、无监督/半监督聚类/社区检测
1.《SEC:More Accurate Clustering Algorithm via Structural Entropy》
Huang J, Feng Q, Wang J, et al. SEC: More Accurate Clustering Algorithm via Structural Entropy[C]//Proceedings of the AAAI Conference on Artificial Intelligence. 2024, 38(11): 12583-12590.
Abstract: As one of the most popular machine learning tools in the field of unsupervised learning, clustering has been widely used in various practical applications. While numerous methods have been proposed for clustering, a commonly encountered issue is that the existing clustering methods rely heavily on local neighborhood information during the optimization process, which leads to suboptimal performance on real-world datasets. Besides, most existing clustering methods use Euclidean distances or densities to measure the similarity between data points. This could constrain the effectiveness of the algorithms for handling datasets with irregular patterns. Thus, a key challenge is how to effectively capture the global structural information in clustering instances to improve the clustering quality. In this paper, we propose a new clustering algorithm, called SEC. This algorithm uses the global structural information extracted from an encoding tree to guide the clustering optimization process. Based on the relation between data points in the instance, a sparse graph of the clustering instance can be constructed. By leveraging the sparse graph constructed, we propose an iterative encoding tree method, where hierarchical abstractions of the encoding tree are iteratively extracted as new clustering features to obtain better clustering results. To avoid the influence of easily misclustered data points located on the boundaries of the clustering partitions, which we call “fringe points”, we propose an iterative pre-deletion and reassignment technique such that the algorithm can delete and reassign the “fringe points” to obtain more resilient and precise clustering results. Empirical experiments on both synthetic and real-world datasets demonstrate that our proposed algorithm outperforms state-of-the-art clustering methods and achieves better clustering performances. On average, the clustering accuracy (ACC) is increased by 1.7% and the normalized mutual information (NMI) by 7.9% compared with the current state-of-the-art (SOTA) algorithm on synthetic datasets. On real-world datasets, our method outperforms other clustering methods with an average increase of 12.3% in ACC and 5.2% in NMI, respectively.
虽然目前已经提出了多种聚类方法,但一个常见的问题是现有的聚类方法在优化过程中严重依赖局部邻域信息,这导致在实际数据集上的性能不佳。此外,大多数现有的聚类方法使用欧几里德距离或密度来衡量数据点之间的相似性,这可能会限制处理具有不规则模式数据集的有效性。因此,一个关键的挑战是如何有效地捕获聚类实例中的全局结构信息以提高聚类质量。本文提出了一种新的聚类算法SEC:
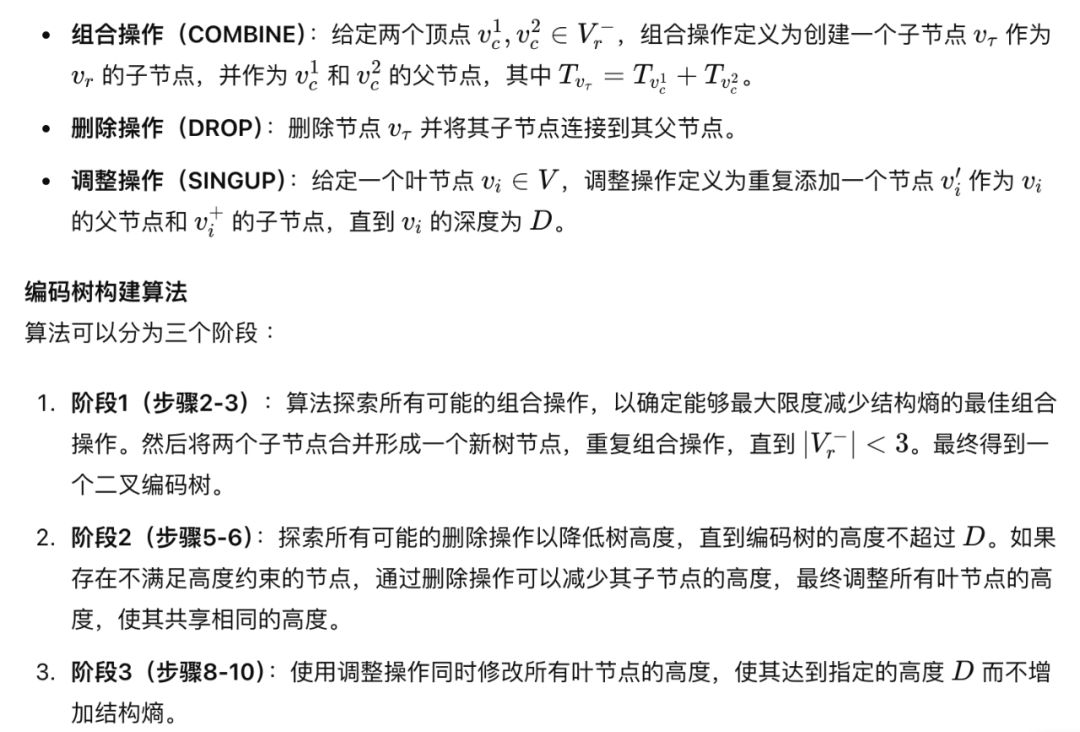
2.《Semi-Supervised Clustering via Structural Entropy with Different Constraint》
Zeng G, Peng H, Li A, et al. Semi-Supervised Clustering via Structural Entropy with Different Constraints[C]//Proceedings of the 2024 SIAM International Conference on Data Mining (SDM). Society for Industrial and Applied Mathematics, 2024: 208-216.Abstract: Semi-supervised clustering techniques have emerged as valuable tools for leveraging prior information in the form of constraints to improve the quality of clustering outcomes. Despite the proliferation of such methods, the ability to seamlessly integrate various types of constraints remains limited. While structural entropy has proven to be a powerful clustering approach with wide-ranging applications, it has lacked a variant capable of accommodating these constraints. In this work, we present Semi-supervised clustering via Structural Entropy (SSE), a novel method that can incorporate different types of constraints from diverse sources to perform both partitioning and hierarchical clustering. Specifically, we formulate a uniform view for the commonly used pairwise and label constraints for both types of clustering. Then, we design objectives that incorporate these constraints into structural entropy and develop tailored algorithms for their optimization. We evaluate SSE on nine clustering datasets and compare it with eleven semi-supervised partitioning and hierarchical clustering methods. Experimental results demonstrate the superiority of SSE on clustering accuracy with different types of constraints. Additionally, the functionality of SSE for biological data analysis is demonstrated by cell clustering experiments conducted on four single-cell RNA-seq datasets.
半监督聚类技术已成为利用约束形式的先验信息来提高聚类结果质量的宝贵工具。尽管此类方法不断涌现,但无缝集成各种类型约束的能力仍然有限。
虽然结构熵已被证明是一种具有广泛应用的强大聚类方法,但它缺乏能够适应这些约束的变体
我们提出了通过结构熵的半监督聚类SSE,输入数据和约束被转换为两个具有相同顶点集的不同图,然后分别通过2D SSE和高维SSE最小化执行半监督划分聚类和半监督层次聚类
统一的约束表示
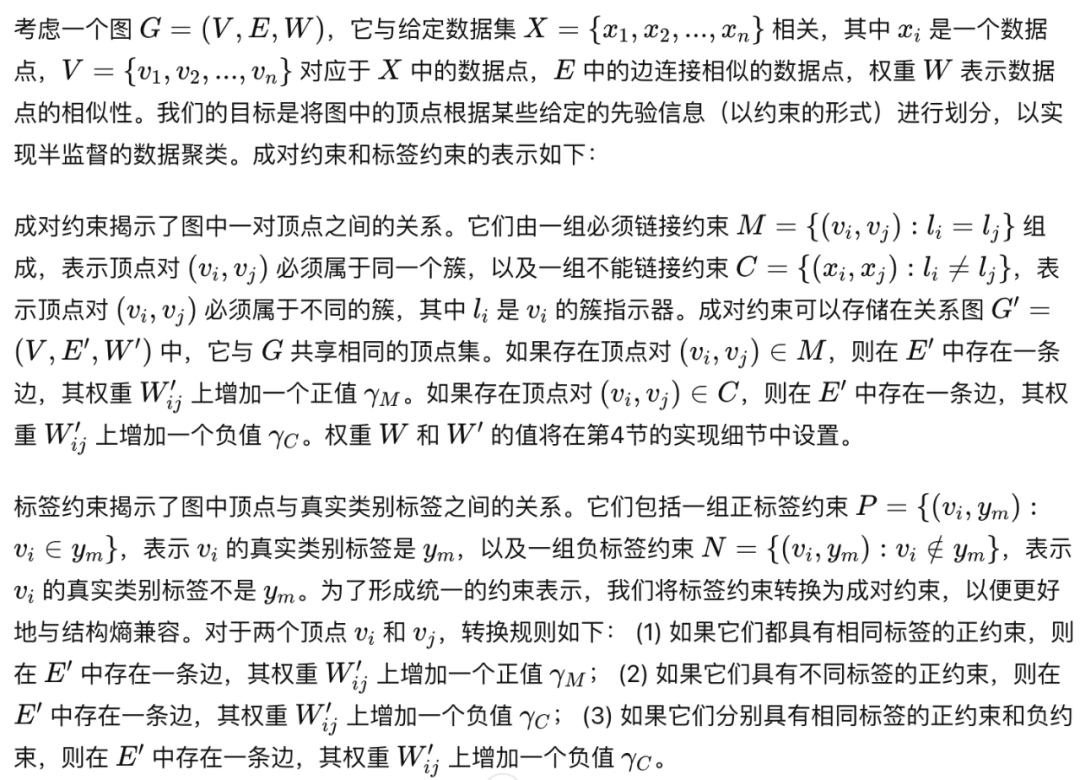
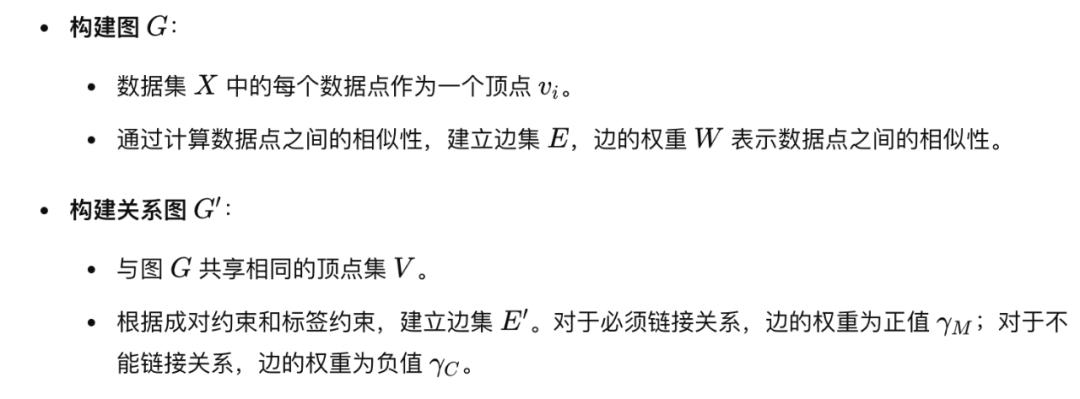
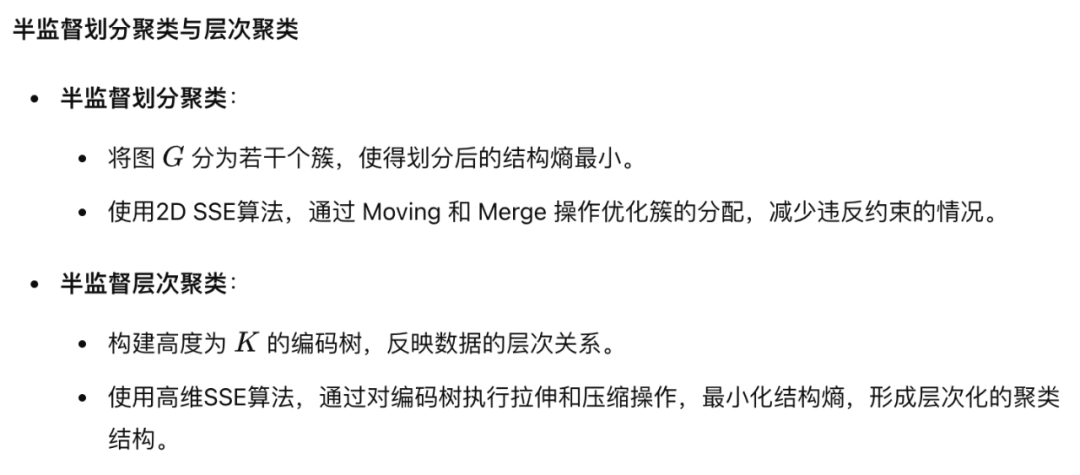
2D 结构熵
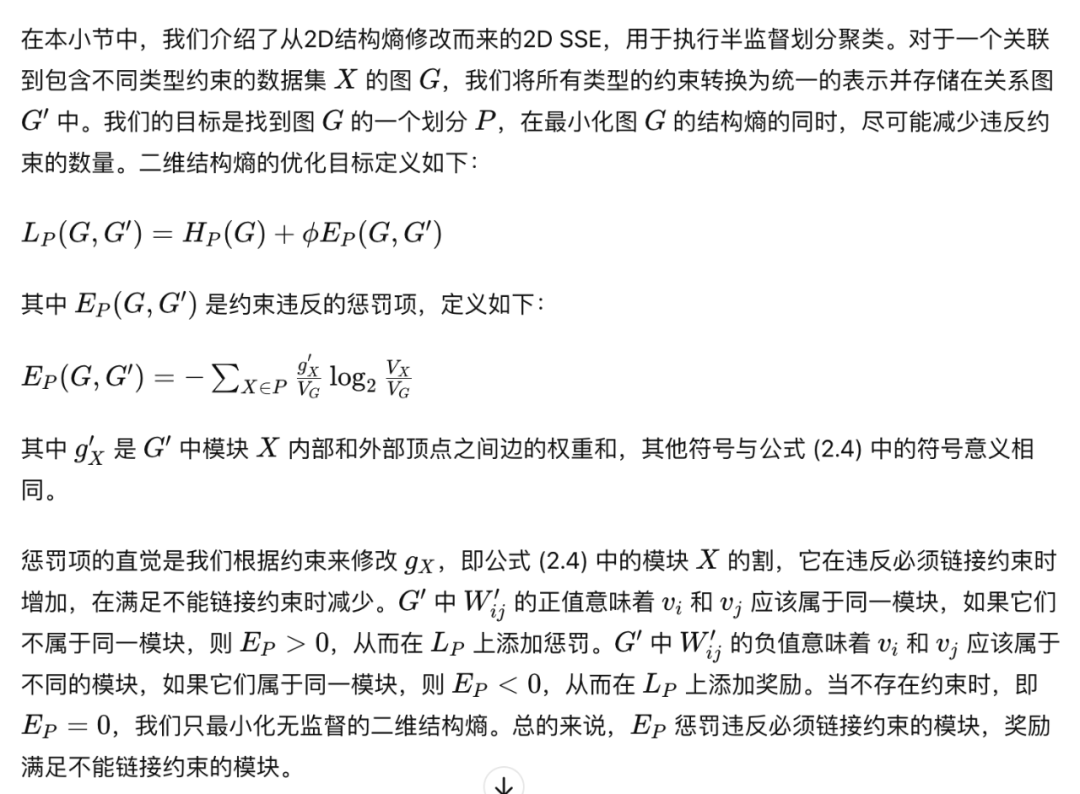
在二维结构熵(2D SSE)最小化过程中,存在两个关键操作:Moving 和 Merge 操作。

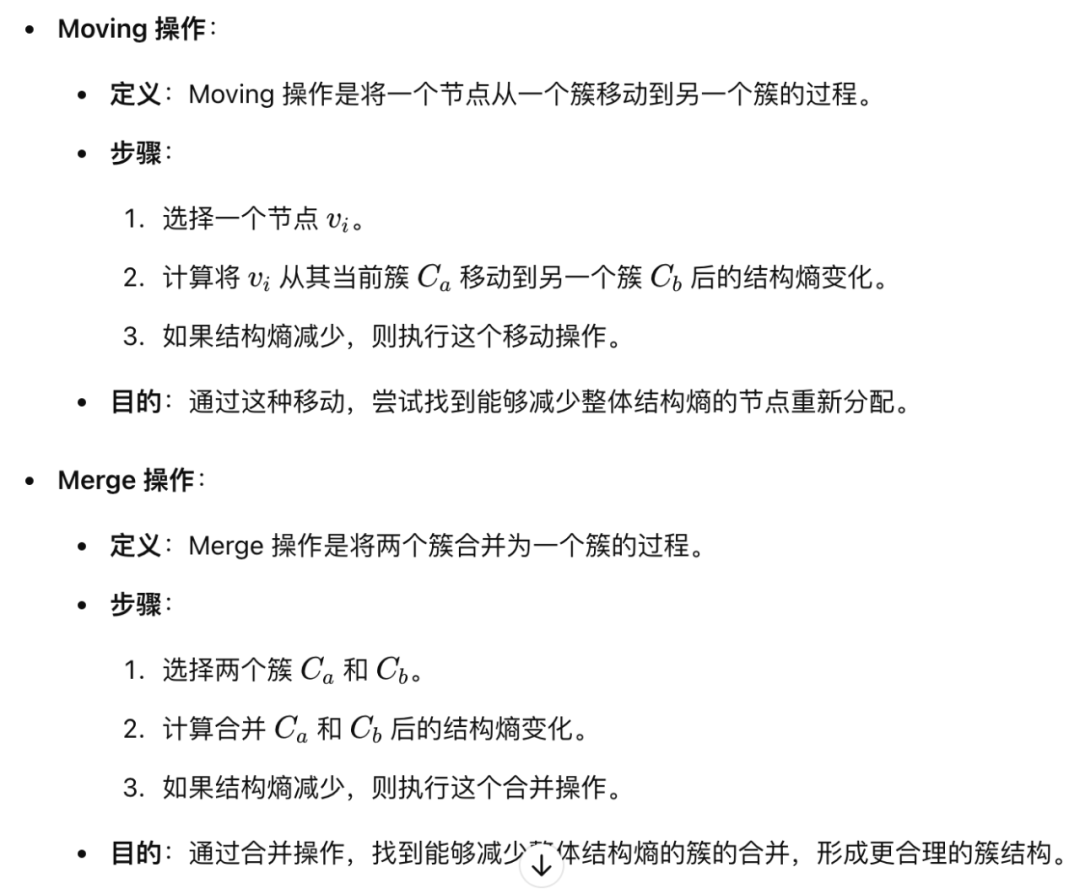
高维结构熵
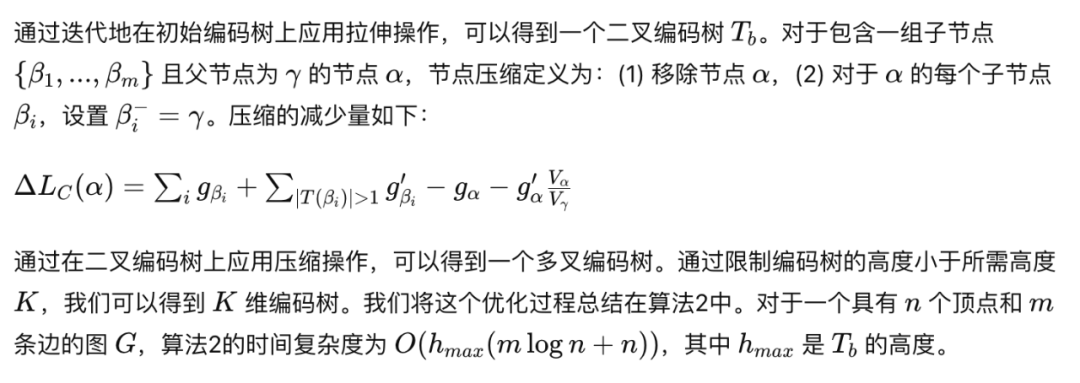
在 9 个聚类数据集上评估 SSE,并与 11 种半监督分区和层次聚类方法进行比较;
实验结果证明了SSE在不同类型约束下的聚类精度方面的优越性;
此外SSE 用于生物数据分析的功能通过在四个单细胞 RNAseq 数据集上进行的细胞聚类实验得到了证明。
SSE框架图。(1)分别由输入数据和约束关系分别构造两个图G和G’。(II)通过合并和移动两个操作实现半监督分区聚类。(III)通过拉伸和压缩两个操作实现半监督分层聚类。
二、社交事件/社交机器人检测
目前,社交机器人检测中使用两类聚类算法:基于特征的聚类算法和基于网络结构的社区检测算法,如K近邻、密度聚类、最大流最小割理论等。这些传统模型表现出单一级别的用户聚类,其中预定的聚类数量可能会影响聚类的效果。而基于神经网络的深度聚类方法缺乏可解释性。此外,所采用的距离测量方法可能并不完全合适。结构信息理论通过构建结构熵编码树并解码社交网络的本质结构,提供了社会用户的自适应分层社区划分。
1.《Hierarchical and Incremental Structural Entropy Minimization for Unsupervised Social Event Detection》
Cao Y, Peng H, Yu Z, et al. Hierarchical and incremental structural entropy minimization for unsupervised social event detection[C]//Proceedings of the AAAI Conference on Artificial Intelligence. 2024, 38(8): 8255-8264.Abstract: As a trending approach for social event detection, graph neural network (GNN)-based methods enable a fusion of natural language semantics and the complex social network structural information, thus showing SOTA performance. However, GNN-based methods can miss useful message correlations. Moreover, they require manual labeling for training and predetermining the number of events for prediction. In this work, we address social event detection via graph structural entropy (SE) minimization. While keeping the merits of the GNN-based methods, the proposed framework, HISEv- ent, constructs more informative message graphs, is unsupervised, and does not require the number of events given a priori. Specifically, we incrementally explore the graph neighborhoods using 1-dimensional (1D) SE minimization to supplement the existing message graph with edges between semantically related messages. We then detect events from the message graph by hierarchically minimizing 2-dimensional (2D) SE. Our proposed 1D and 2D SE minimization algorithms are customized for social event detection and effectively tackle the efficiency problem of the existing SE minimization algorithms. Extensive experiments show that HI-SEvent consistently outperforms GNN-based methods and achieves the new SOTA for social event detection under both closed- and open-set settings while being efficient and robust.社交事件检测作为舆情挖掘、假新闻检测等的基础,越来越受到工业界和学术界的关注。现有研究通常将社交事件检测任务形式化为从社交媒体序列中提取相关消息簇消息。近年来,基于图神经网络的社会事件检测研究蓬勃发展,然而现有GNN方法在构建消息图时,通常仅仅考虑共享属性的消息之间的连接,忽略了语义相近但没有共同属性的消息之间的相关性【挑战1】。此外,这些模型的 GNN 组件需要监督训练并预先确定用于预测的事件总数【挑战2】。最近的基于 GNN 的方法开始采用对比学习、归纳学习和伪标签生成来缓解对标签的依赖。然而,对于初始培训和定期维护,手动标记仍然是必要的。
本文通过图结构熵最小化来解决社交事件检测。在保留基于 GNN 的方法的优点的同时,所提出的框架 HISEvent 构造了信息更丰富的消息图,是无监督的,并且不需要先验给定事件的数量。
解决挑战1:使用增量一维 (Incremental 1D) SE最小化逐步探索图邻域,以用语义相关消息之间的边来补充现有的消息图
初始化:
输入:消息图节点集V;
输出:基于语义相似性的边集;
初始化一个空的边集SEs和消息节点的嵌入表示{hmi}。
嵌入与排序:
使用预训练语言模型对消息节点进行嵌入,获取每个消息节点的表示;
对于每个节点i,将其所有其他节点按照与i的余弦相似度从高到低排序,得到邻居节点集合neighbmi。
构建初始边集:
从每个节点的邻居中选取第一个节点作为初始边集E的一部分;
计算初始的1D SE H(1)(G),并将其添加到SEs中。
增量式边集扩展:
逐步将每个节点的第个最近邻居加入边集中,更新边集E;
计算更新后的1D SE H(1)(G),并将其添加到SEs中;
重复上述过程,直到找到第一个稳定点,即(k-1)处的1D SE比其前后值都小。
确定最终边集:
相关公式:
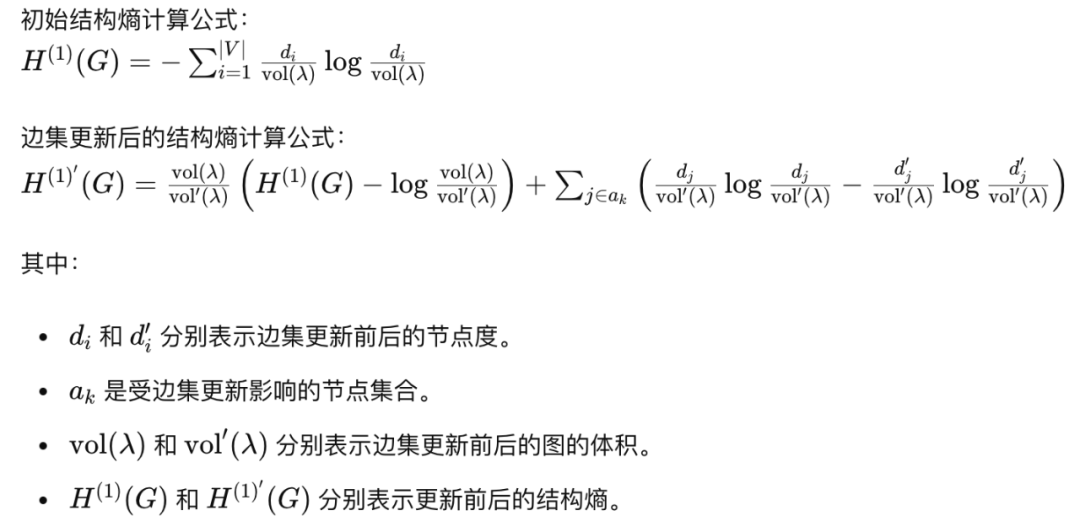
解决挑战2:通过分层最小化二维 (Hierarchical 2D) SE 来检测消息图中的事件
初始化:
输入:消息图G = (V, E),子图大小n;
输出:节点集V的分区P;
初始时每个消息节点都是一个独立的簇。
层次化2D SE最小化:
将当前簇集P划分为大小为n的子集Ps;
对于每个子集Ps,合并子集中所有的簇,形成子图G';
为G'构建初始编码树T',其中每个簇作为一个内部节点,每个消息作为一个叶节点;
对子图G'运行基本的2D SE最小化算法,得到新的簇P';
将新的簇P'加入到全局簇集P中。
迭代更新:
重复上述步骤,直到所有消息节点都被考虑在内;
如果在某次迭代中没有簇被合并,则增加子图大小n,以便在下一次迭代中考虑更多的簇。
总结:层次化2D MrSE最小化VS普通2D MrSE最小化算法。
(1)处理方式:
普通2D:一次性考虑整个图G'。
层次化2D:将图分成多个子图,逐个处理这些子图。
(2)算法流程:
(3)时间复杂度:
(4)适用场景:
普通2D:适合中小规模图;
层次化2D:特别适合大规模和稠密图。
(5) 参数:
普通2D:不需要额外参数;
层次化2D:需要设置子图大小n。
大量实验表明,HISEvent 始终优于基于 GNN 的方法,并在封闭集和开放集设置下实现了社交事件检测的新 SOTA,同时高效且稳健。
HISEvent框架图。A和B分别是消息图的构建和划分过程。初始消息图A.2是通过链接共享公共属性的社交消息(A.1)来构建的。进一步添加基于语义相似度的边集
,得到最终的消息图(A.3)。B.2展示了我们提出的分层二维SE最小化算法,该算法从子图(
G)中重复检测聚类(
P)。B.1展示了如何通过普通2D SE最小化在单个子图中检测聚类。B.3为检测到的社会事件。
2.《Adversarial Social bots Modeling Based on Structural Information Principles》
Zeng X, Peng H, Li A. Adversarial socialbots modeling based on structural information principles[C]//Proceedings of the AAAI Conference on Artificial Intelligence. 2024, 38(1): 392-400.Abstract: The importance of effective detection is underscored by the fact that socialbots imitate human behavior to propagate mis-information, leading to an ongoing competition between socialbots and detectors. Despite the rapid advancement of reactive detectors, the exploration of adversarial socialbot modeling remains incomplete, significantly hindering the development of proactive detectors. To address this issue, we propose a mathematical Structural Information principles-based Adversarial Socialbots Modeling framework, namely SIASM, to enable more accurate and effective modeling of adversarial behaviors. First, a heterogeneous graph is presented to integrate various users and rich activities in the original social network and measure its dynamic uncertainty as structural entropy. By minimizing the high-dimensional structural entropy, a hierarchical community structure of the social network is generated and referred to as the optimal encoding tree. Secondly, a novel method is designed to quantify influence by utilizing the assigned structural entropy, which helps reduce the computational cost of SIASM by filtering out uninfluential users. Besides, a new conditional structural entropy is defined between the socialbot and other users to guide the follower selection for network influence maximization. Extensive and comparative experiments on both homogeneous and heterogeneous social networks demonstrate that, compared with state-of-the-art baselines, the proposed SIASM framework yields substantial performance improvements in terms of network influence (up to 16.32%) and sustainable stealthiness (up to 16.29%) when evaluated against a robust detector with 90% accuracy.
提出一个异构图来整合原始社交网络中的各种用户和丰富的活动,并将其动态不确定性度量为结构熵。通过最小化高维结构熵,生成社交网络的分层社区结构,称为最优编码树。


设计了一种利用分配的结构熵来量化影响力的新方法,这有助于通过过滤掉无影响力的用户来降低 SIASM 的计算成本。
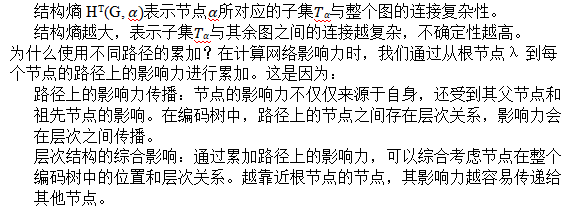
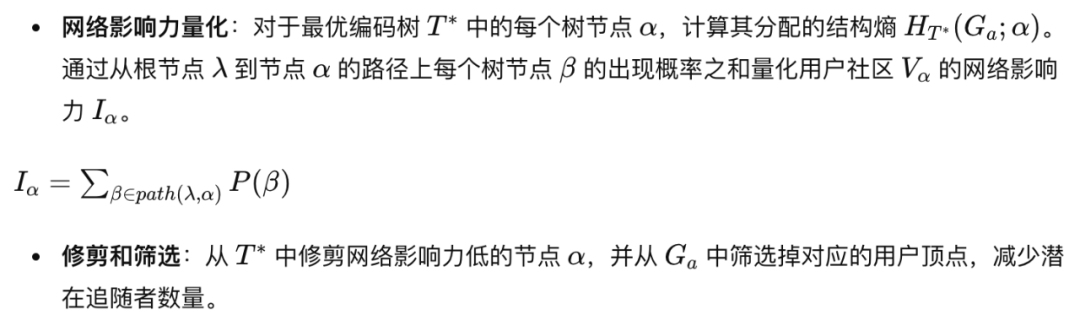
在社交机器人和每个用户节点之间定义一个条件结构熵,以指导追随者选择以实现最大化社交机器人的网络影响力。
(条件结构熵反映消息从机器人传播到u的确定性,熵越小,确定性越高)
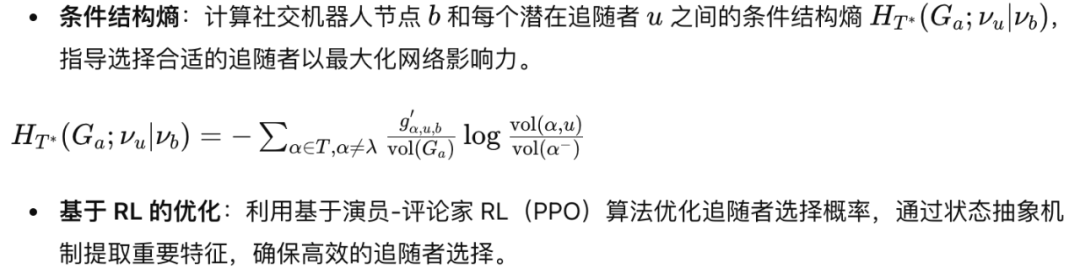
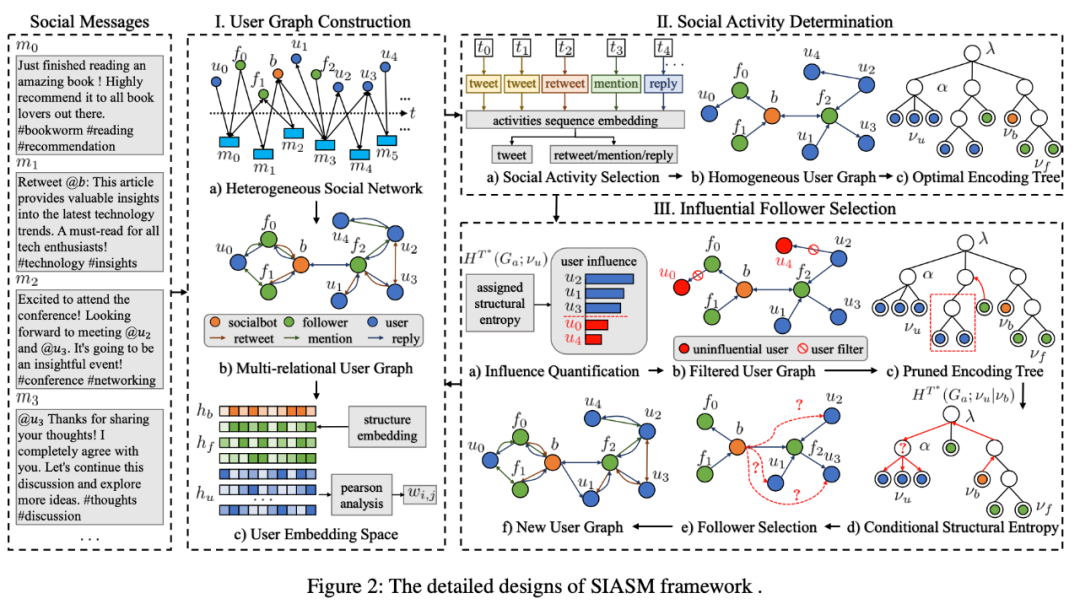
3.《SeBot: Structural Entropy Guided Multi-View Contrastive Learning for Social Bot Detection》
Yang Y, Wu Q, He B, et al. SeBot: Structural Entropy Guided Multi-View Contrastive Learning for Social Bot Detection[J]. 2024 KDDAbstract: Recent advancements in social bot detection have been driven by the adoption of Graph Neural Networks. The social graph, constructed from social network interactions, contains benign and bot accounts that influence each other. However, previous graph-based detection methods that follow the transductive message-passing paradigm may not fully utilize hidden graph information and are vulnerable to adversarial bot behavior. The indiscriminate message passing between nodes from different categories and communities results in excessively homogeneous node representations, ultimately reducing the effectiveness of social bot detectors. In this paper, we propose SeBot, a novel multi-view graph-based con- trastive learning-enabled social bot detector. In particular, we use structural entropy as an uncertainty metric to optimize the entire graph’s structure and subgraph-level granularity, revealing the implicitly existing hierarchical community structure. And we design an encoder to enable message passing beyond the homophily assumption, enhancing robustness to adversarial behaviors of social bots. Finally, we employ multi-view contrastive learning to maximize mutual information between different views and enhance the detection performance through multi-task learning. Experimental results demonstrate that our approach significantly improves the performance of social bot detection compared with SOTA methods.图神经网络的采用推动了社交机器人检测的最新进展。社交图谱由社交网络交互构建而成,包含相互影响的良性帐户和机器人帐户
然而,以前遵循传导消息传递范式的基于图的检测方法可能无法充分利用隐藏的图信息,并且容易受到对抗性机器人行为的影响【挑战1:如何充分利用隐藏在图结构中的层次信息?——主要侧重于聚合原始图上的节点级信息,忽略了高阶图结构信息的综合利用。因此,生成的表示仅捕获低阶图信息而缺乏高阶语义信息】
来自不同类别和社区的节点之间不加区别地传递消息会导致节点表示过于同质,最终降低社交机器人检测器的有效性【挑战2:如何处理机器人故意与人类交互以逃避检测的对抗行为?】
本文提出了 SeBot,一种新颖的基于多视图的对比学习的社交机器人检测器
使用结构熵作为不确定性度量来优化整个图的结构和子图级粒度,揭示隐含存在的分层社区结构;
设计编码器,使消息传递超越同质假设,增强社交机器人对抗行为的鲁棒性;
采用多视图对比学习来最大化不同视图之间的互信息,并通过多任务学习来增强检测性能。

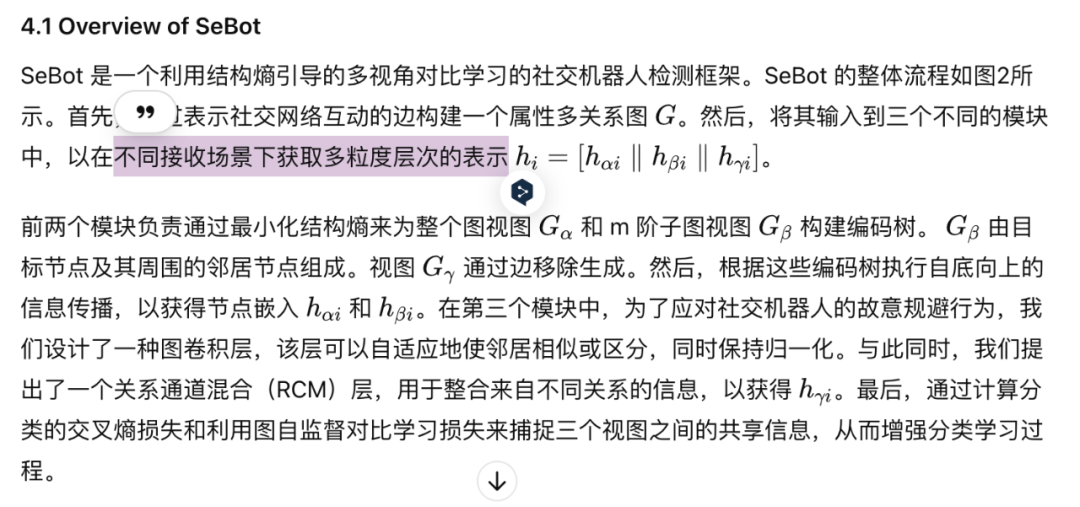
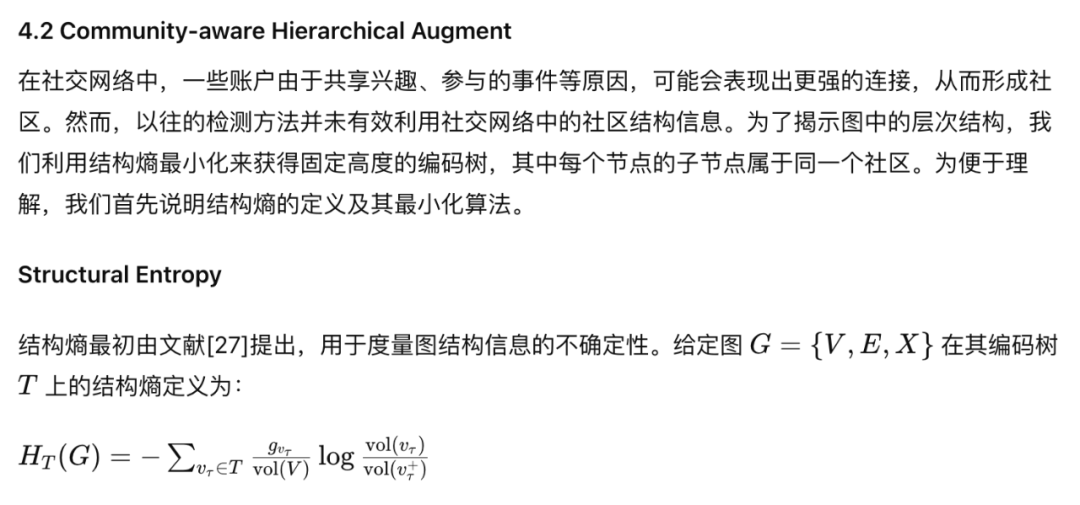
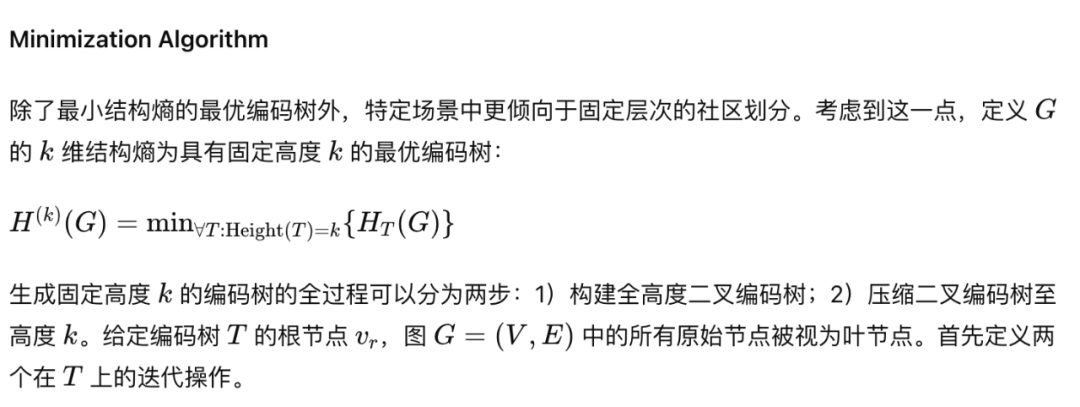
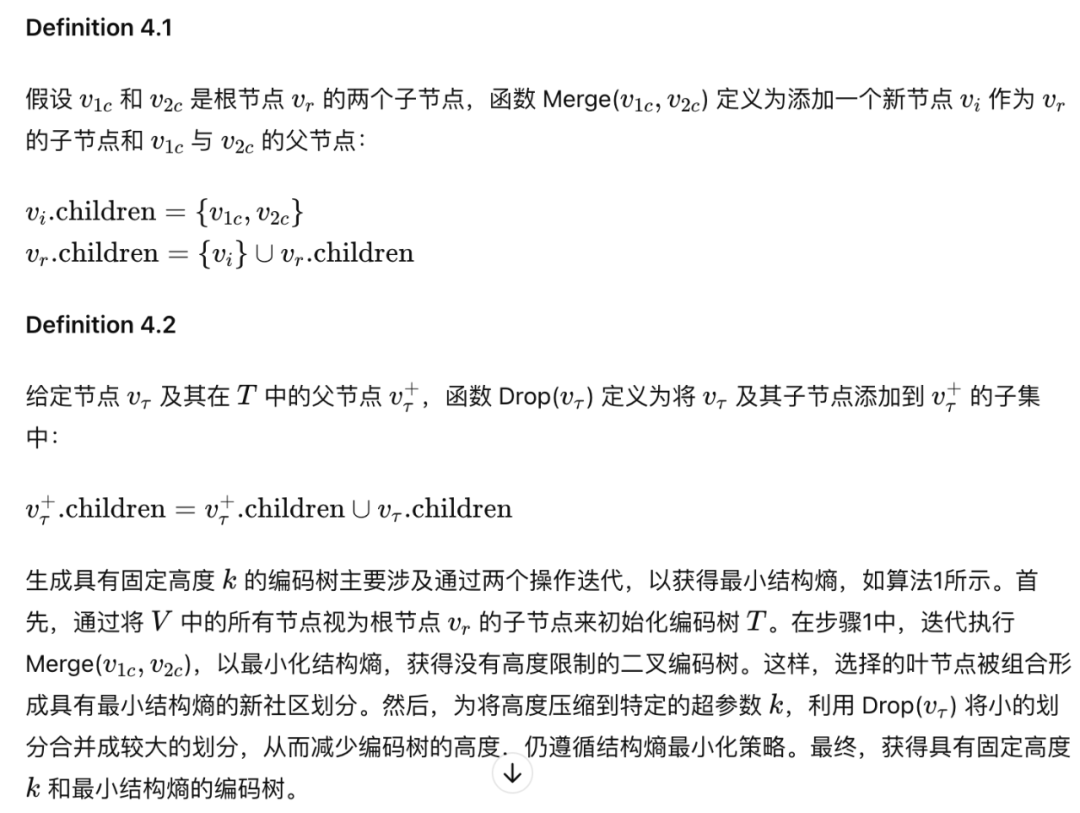
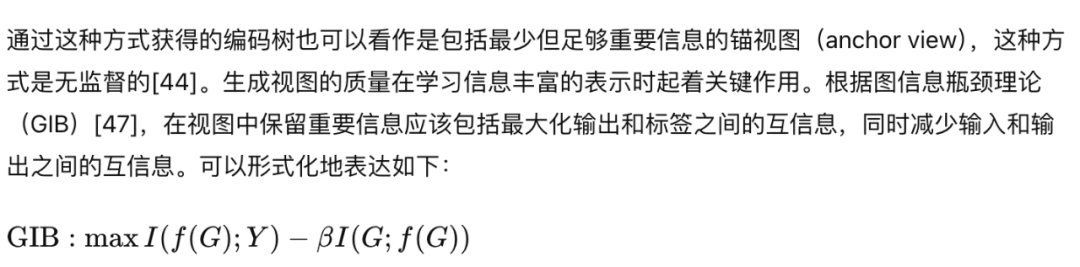
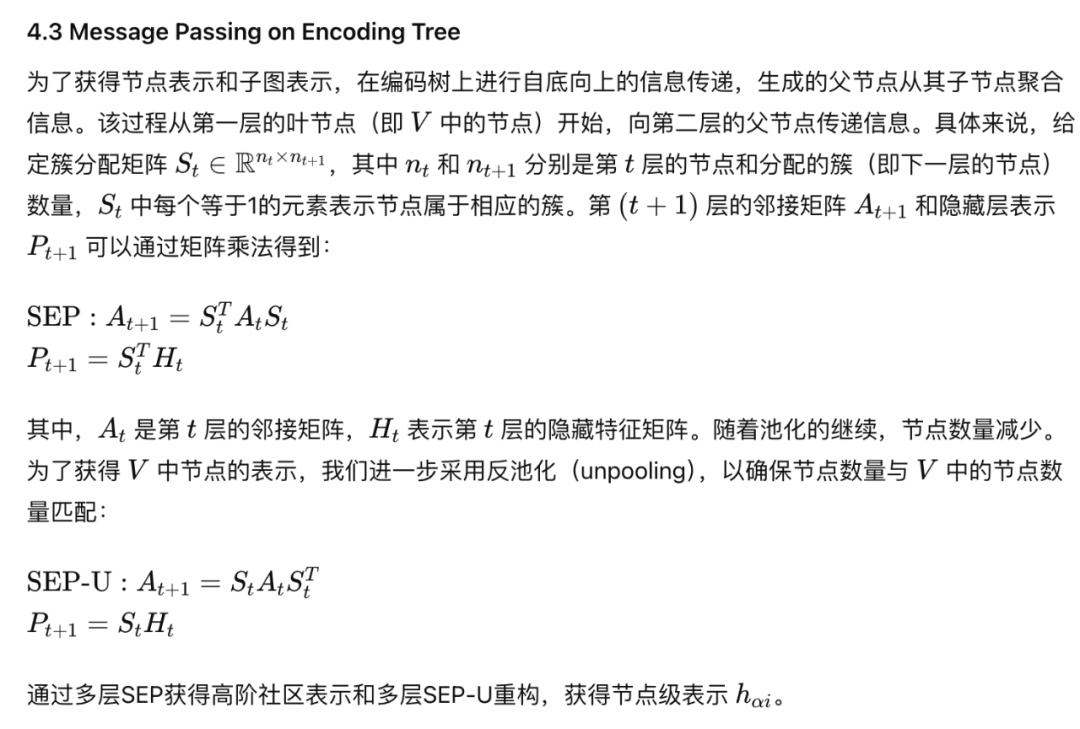
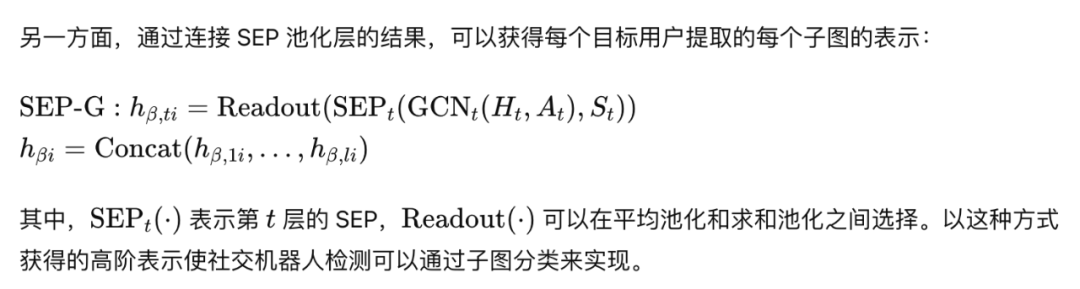
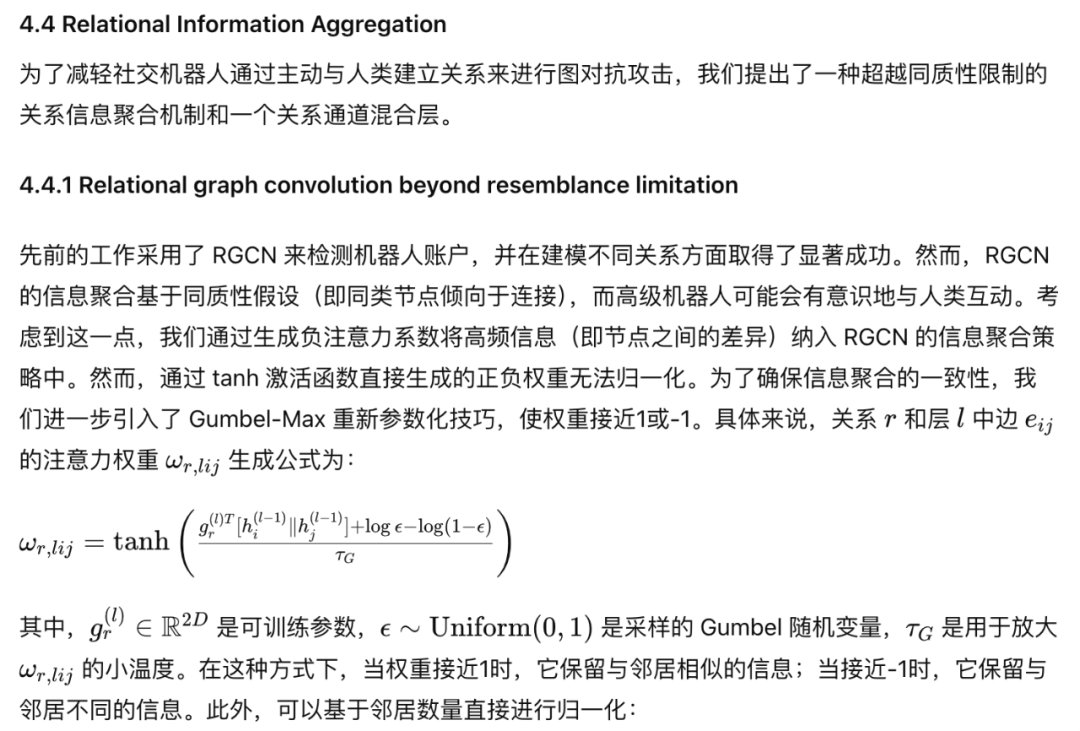
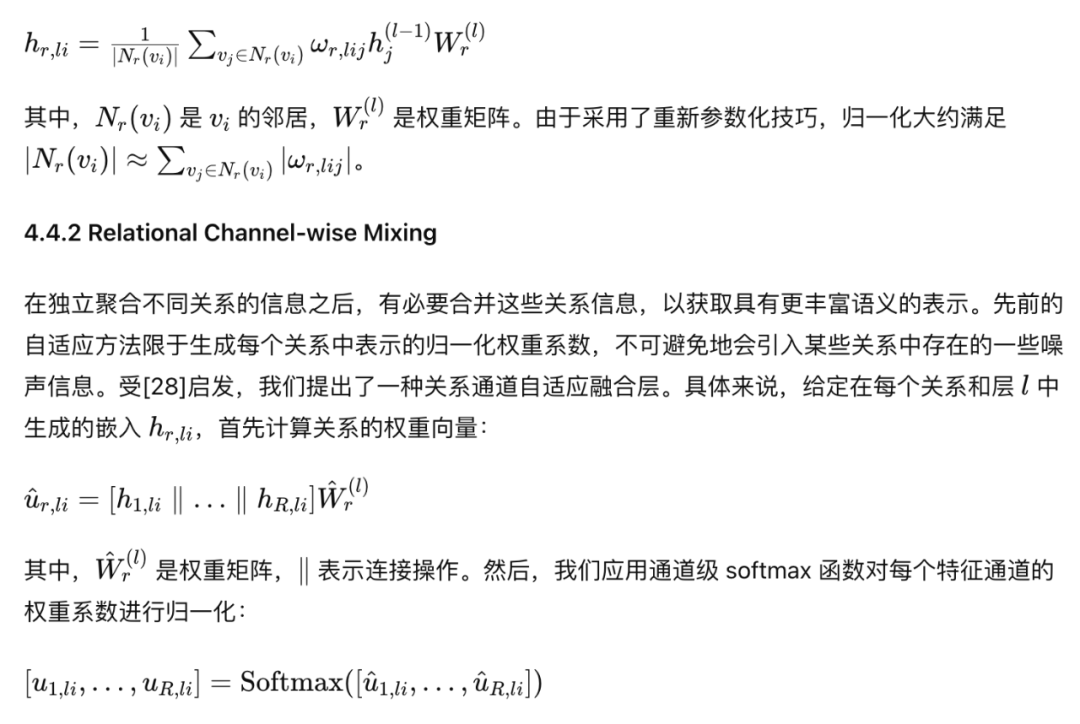
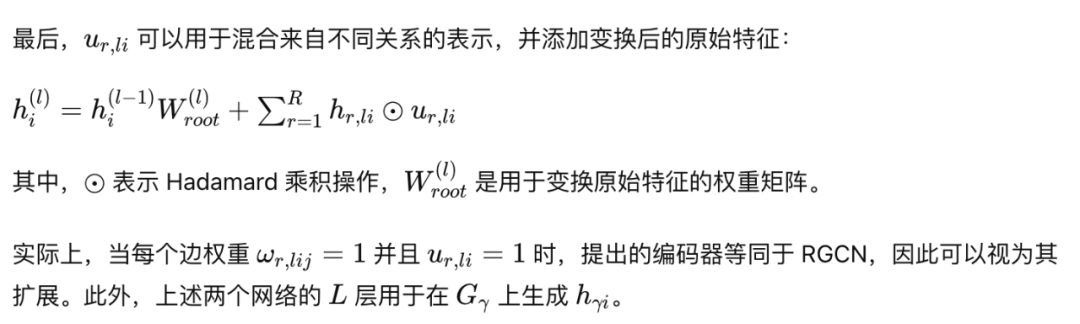
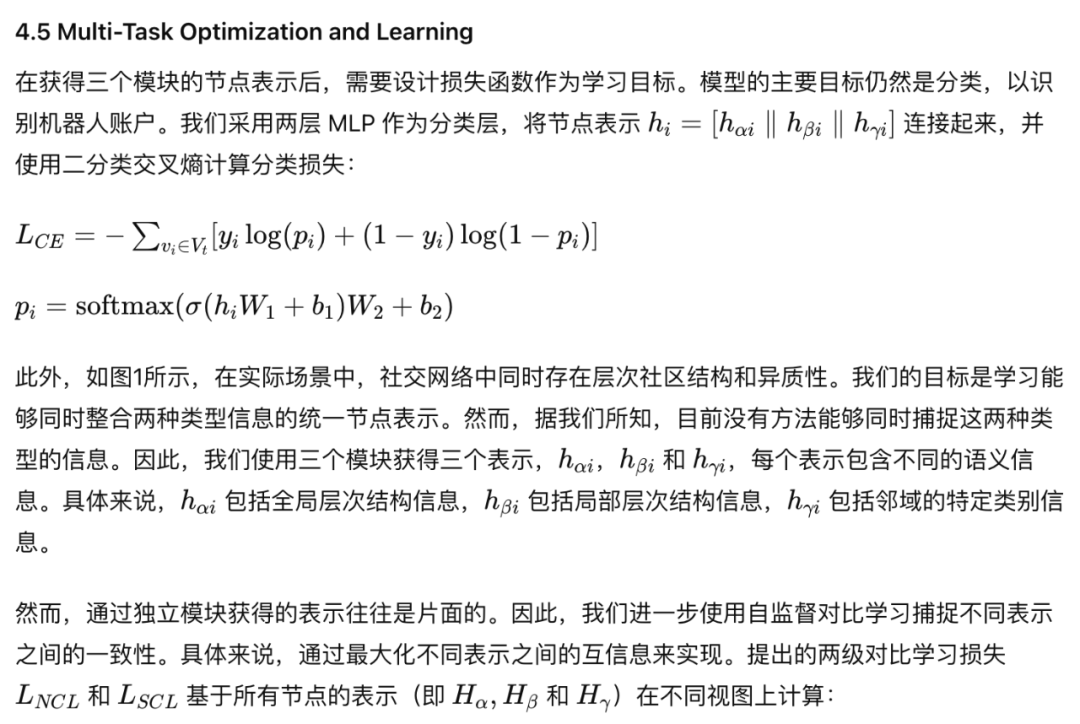
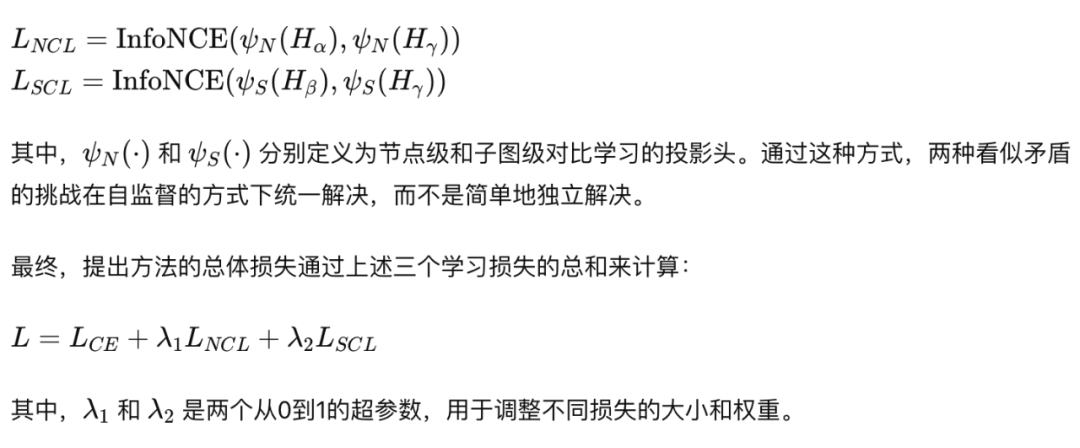
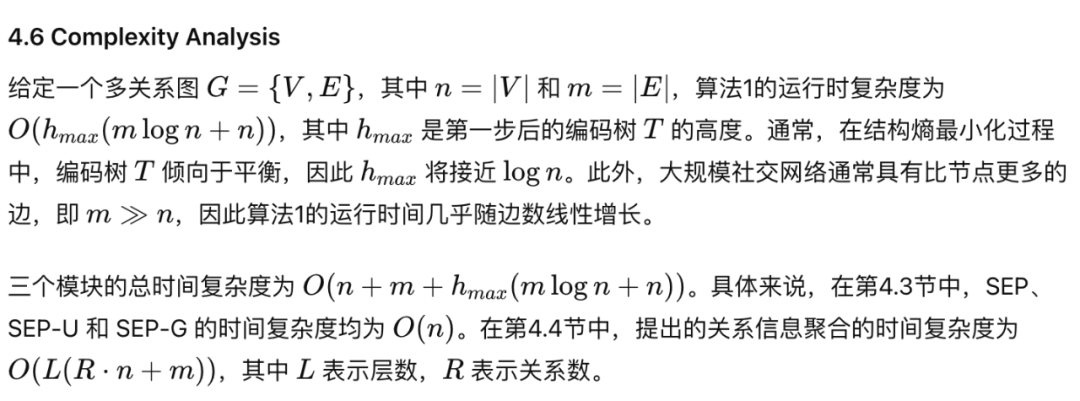
我们提出的框架SeBot的概述,它主要由三个模块组成:1)节点级编码树生成和自下而上的消息传递;2)子图级编码树生成和消息传递;3)超越同质性的关系信息聚合;4)随后根据获得的树类型表示计算对比学习损失和分类损失。
4. 《Unsupervised Social Bot Detection via Structural Information Theory》
Hao Peng, Jingyun Zhang, Xiang Huang, Zhifeng Hao, Angsheng Li, Zhengtao Yu, and Philip S. Yu. 2024. Unsupervised Social Bot Detection via Structural Information Theory. ACM Trans. Inf. Syst. Just Accepted (April 2024). https://doi.org/10.1145/3660522Abstract: Research on social bot detection plays a crucial role in maintaining the order and reliability of information dissemination while increasing trust in social interactions. The current mainstream social bot detection models rely on black-box neural network technology, e.g., Graph Neural Network, Transformer, etc., which lacks interpretability. In this work, we present UnDBot, a novel unsupervised, interpretable, yet effective and practical framework for detecting social bots. This framework is built upon structural information theory. We begin by designing three social relationship metrics that capture various aspects of social bot behaviors: Posting Type Distribution, Posting Influence, and Follow-to-follower Ratio. Three new relationships are utilized to construct a new, unified, and weighted social multi-relational graph, aiming to model the relevance of social user behaviors and discover long-distance correlations between users. Second, we introduce a novel method for optimizing heterogeneous structural entropy. This method involves the personalized aggregation of edge information from the social multi-relational graph to generate a two-dimensional encoding tree. The heterogeneous structural entropy facilitates decoding of the substantial structure of the social bots network and enables hierarchical clustering of social bots. Thirdly, a new community labeling method is presented to distinguish social bot communities by computing the user’s stationary distribution, measuring user contributions to network structure, and counting the intensity of user aggregation within the community. Compared with ten representative social bot detection approaches, comprehensive experiments demonstrate the advantages of effectiveness and interpretability of UnDBot on four real social network datasets.目前主流的社交机器人检测模型依赖黑盒神经网络技术,例如图神经网络、Transformer等,缺乏可解释性。本文提出了一种新颖的无监督、可解释、有效且实用的框架,用于检测社交机器人。该框架建立在结构信息理论的基础上。
首先,设计三个社交关系指标来捕获社交机器人行为的各个方面:发布类型分布、发布影响力和关注者比率,利用这三种新的关系构建一个新的、统一的、加权的社交多关系图。这些关系代表用户行为特征的相似性, 与依赖于关注和转发等直接用户交互的传统图不同,这种多关系图更加重视用户之间社交行为的隐藏共性。【挑战1:如何建模社交用户网络来服务社交机器人聚类任务?】
其次,开发了一种优化异质结构熵的新方法——最小化多关系图的结构熵(如下图)。该方法涉及对社交多关系图的边缘信息进行个性化聚合以生成二维编码树,实现社交机器人的分层聚类【挑战2:如何实现社交用户的自适应层次聚类?】
与其他聚类方法相比,编码树聚类方法在选择图上群落的数量和大小方面具有更强的适应性。然而,这种方法仅限于同质图和简单图。为了解决在多关系图上构建编码树和划分社区的挑战,我们首先扩展了结构熵的概念,以包含定义的多关系社交图。然后,构造多关系图的最优编码树,对社会用户群体进行有效划分。
对于多关系图,定义如下变量的综合权重表示:
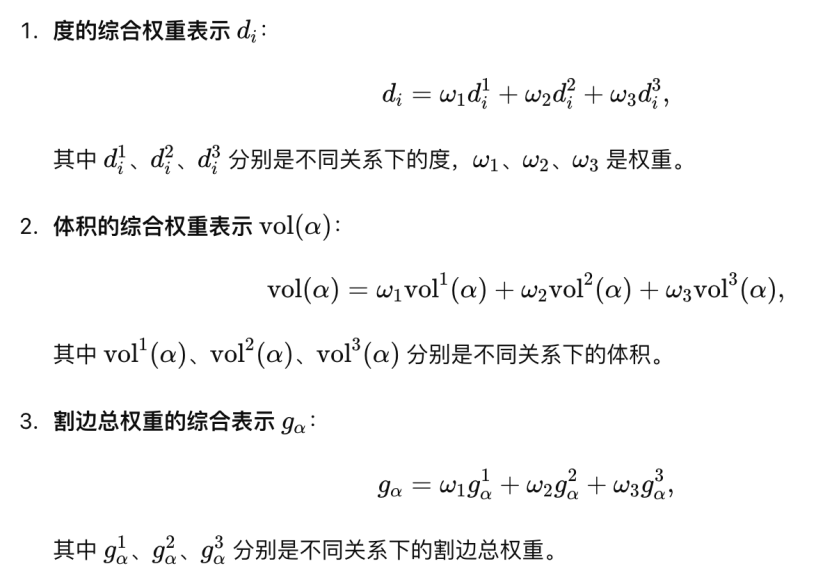
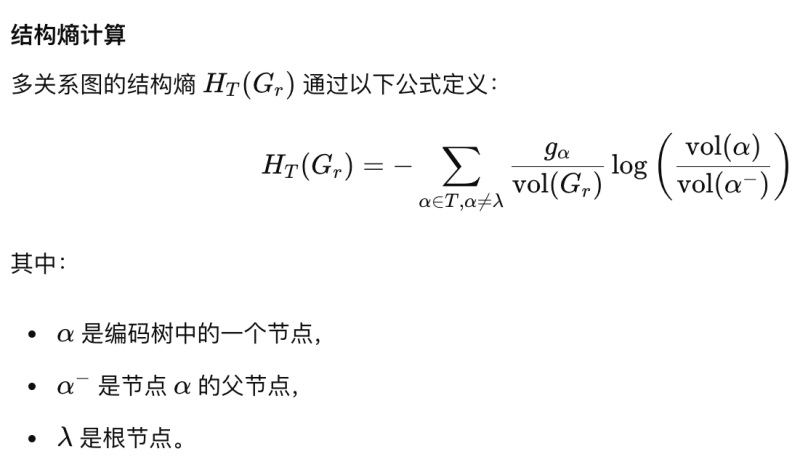
优化编码树:优化编码树的构建涉及一种贪婪搜索策略,本文利用合并(Merge)操作符,该操作符将同一父节点下的两个子树合并为一个子树。合并过程被视为将小社区合并为大社区,从而形成具有最小熵的层次结构。
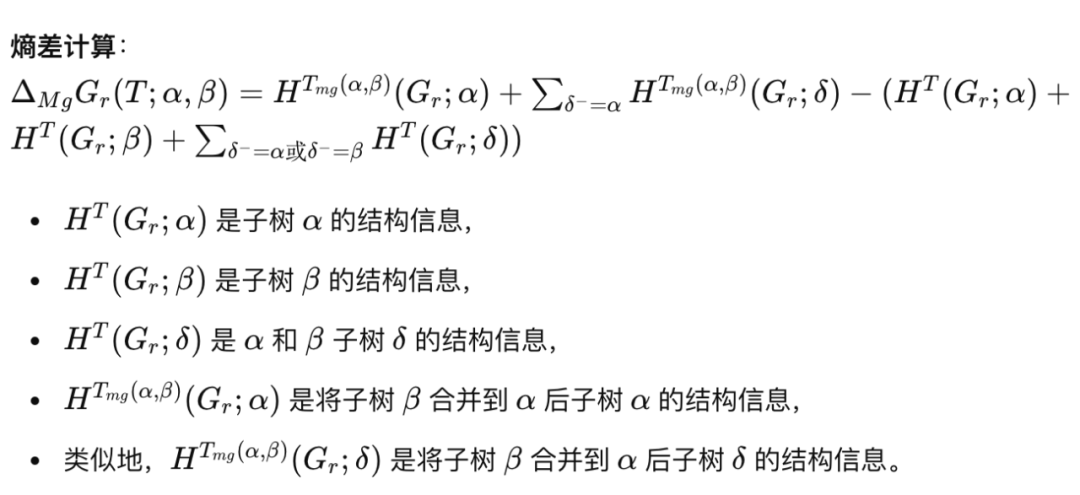
最后,提出了一种新的社区标记方法,结合社区影响力Multirank指标和凝聚力来识别社交机器人社区。编码树上社区节点的熵用于量化社区凝聚力。将这两个指标结合,社交机器人社区可以与正常人类社区区分。【挑战3:如何识别社交用户群体并实现二元分类?(多关系图的编码树只能达到对用户进行聚类的效果。它无法直接将用户分为两个大型社交机器人和人类社区)】
与现有的无监督社交机器人检测模型和基于无监督网络表示学习的模型相比,UnDBot 在四个真实社交网络数据集上的有效性和可解释性的优势。
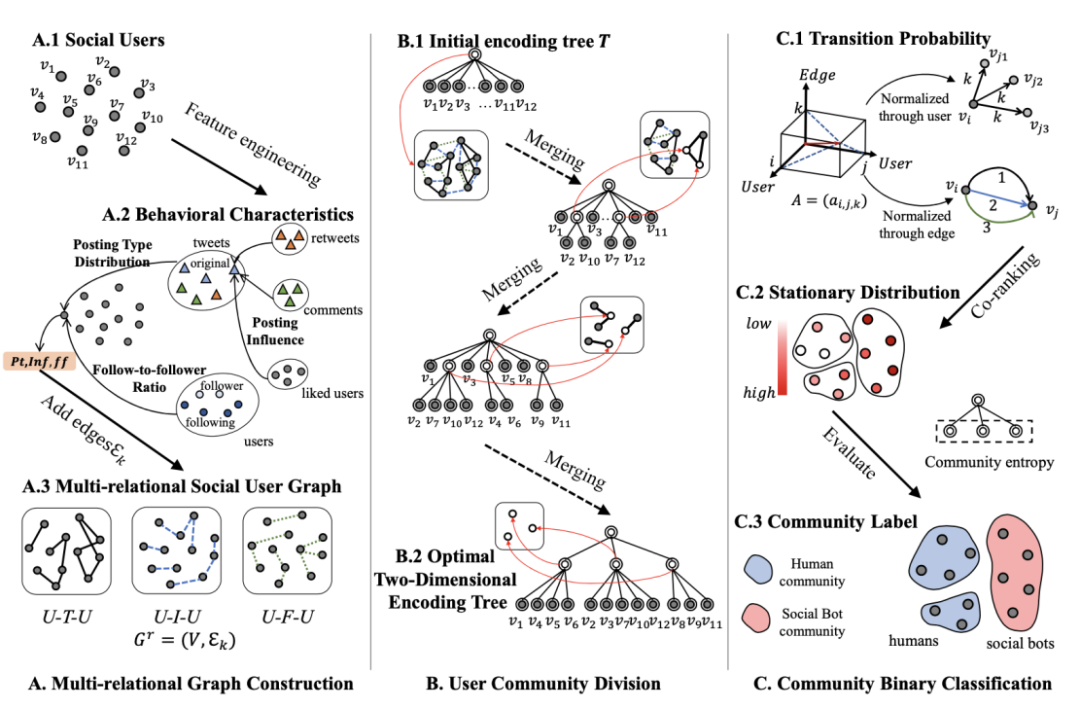
三、角色识别
1.《Effective and Stable Role-Based Multi-Agent Collaboration by Structural Information Principles》
Zeng X, Peng H, Li A. Effective and stable role-based multi-agent collaboration by structural information principles[C]//Proceedings of the AAAI conference on artificial intelligence. 2023, 37(10): 11772-11780.Abstract: Role-based learning is a promising approach to improving the performance of Multi-Agent Reinforcement Learning (MARL). Nevertheless, without manual assistance, current role-based methods cannot guarantee stably discovering a set of roles to effectively decompose a complex task, as they assume either a predefined role structure or practical experience for selecting hyperparameters. In this article, we propose a mathematical Structural Information principles-based Role Discovery method, namely SIRD, and then present a SIRD optimizing MARL framework, namely SR-MARL, for multi-agent collaboration. The SIRD transforms role discovery into a hierarchical action space clustering. Specifically, the SIRD consists of structuralization, sparsification, and optimization modules, where an optimal encoding tree is generated to perform abstracting to discover roles. The SIRD is agnostic to specific MARL algorithms and flexibly integrated with various value function factorization approaches. Empirical evaluations on the StarCraft II micromanagement benchmark demonstrate that, compared with state-of-the-art MARL algorithms, the SR-MARL framework improves the average test win rate by 0.17%, 6.08%, and 3.24%, and reduces the deviation by 16.67%, 30.80%, and 66.30%, under easy, hard, and super hard scenarios.基于角色的学习是提高多智能体强化学习(MARL)性能的一种有前途的方法;
然而,如果没有人工帮助,当前基于角色的方法无法保证稳定地发现一组角色来有效分解复杂的任务,因为它们假设预先定义的角色结构或选择超参数的实践经验;
我们提出了一种基于数学结构信息原理的角色发现方法,即 SIRD,然后提出了一种用于多智能体协作的 SIRD 优化 MARL 框架,即 SR-MARL。
SIRD 将角色发现转变为分层操作空间聚类;
SIRD 由结构化(Structuralization)、稀疏化(Sparsification)和优化模块(Optimization)组成,其中生成最优编码树来执行抽象以发现角色。
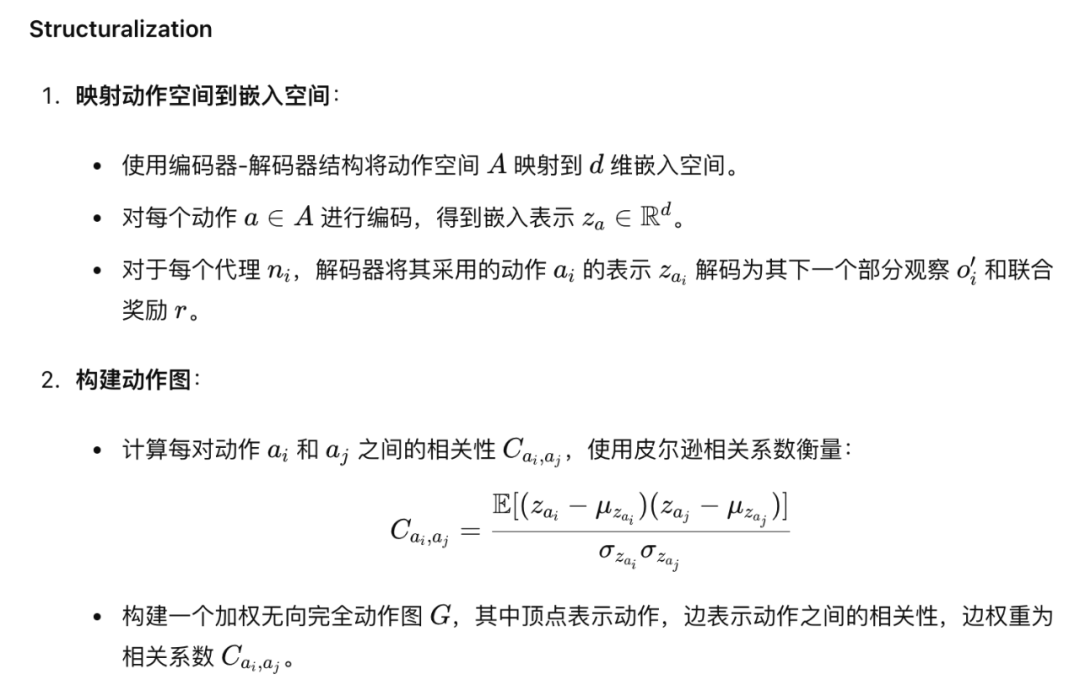
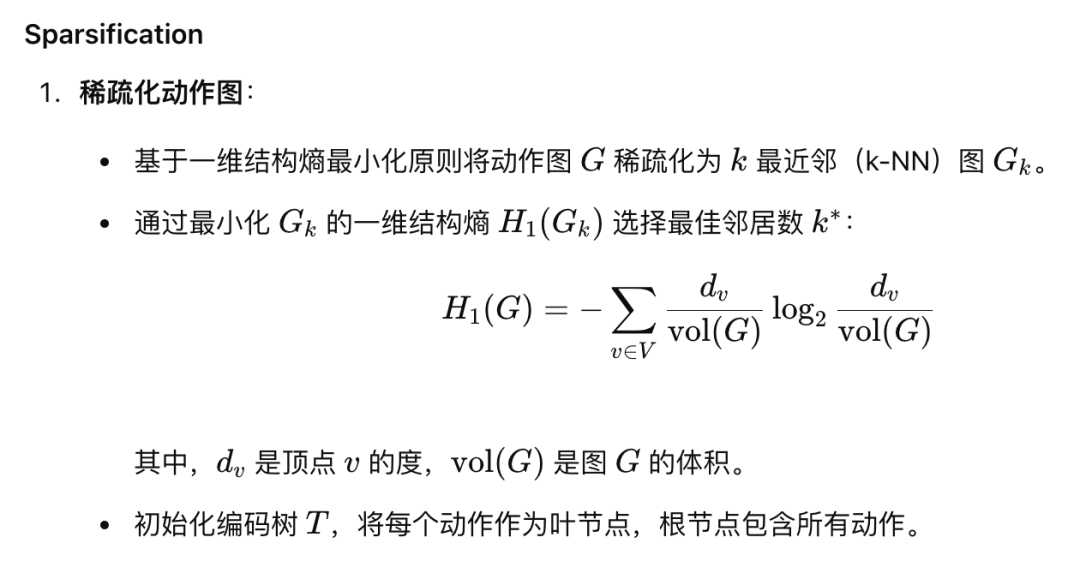
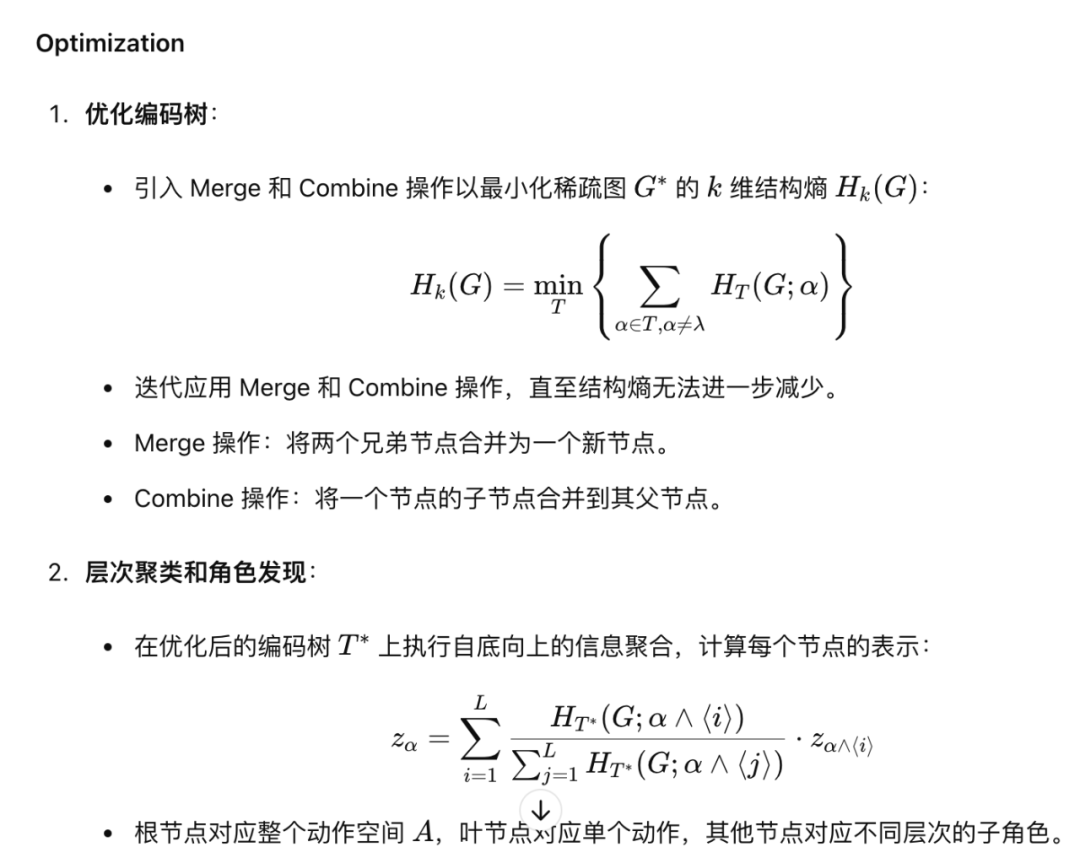
SIRD 与特定的 MARL 算法无关,并且可以与各种值函数分解方法灵活集成。
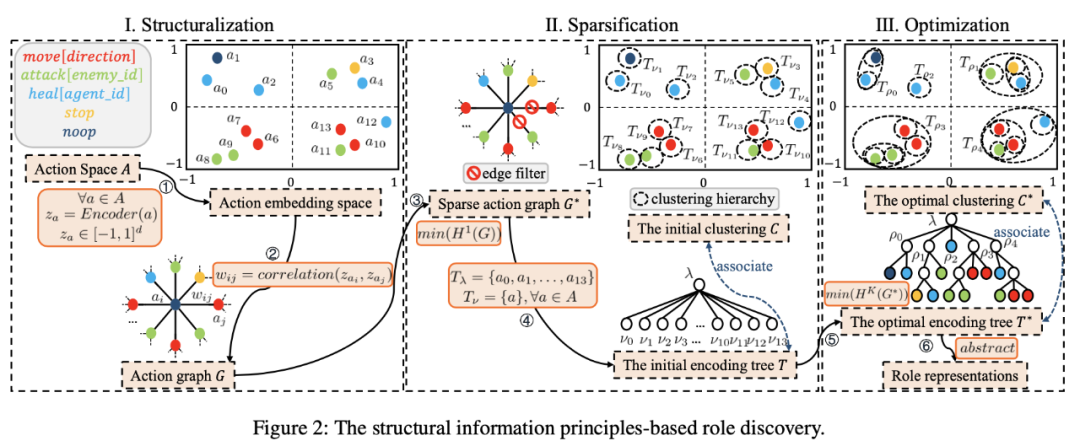
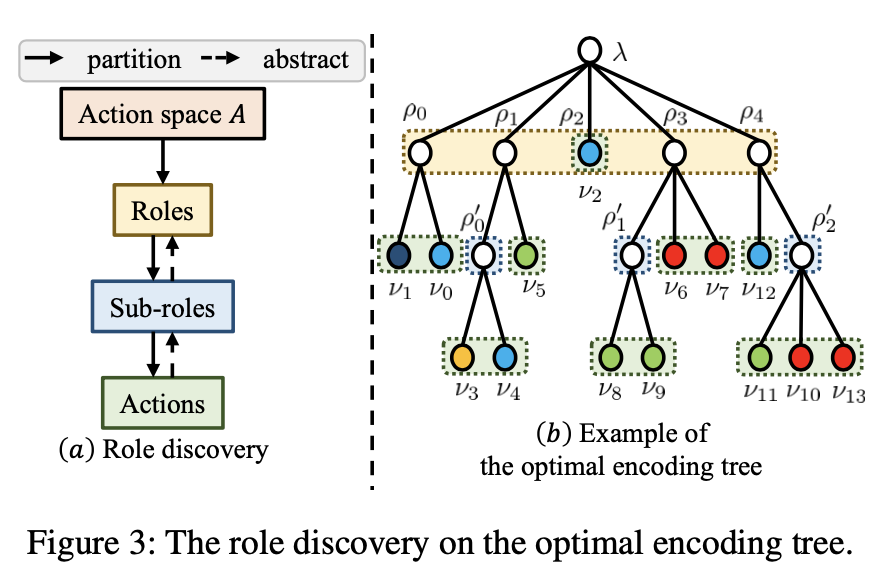
数据派研究部成立于2017年初,以兴趣为核心划分多个组别,各组既遵循研究部整体的知识分享和实践项目规划,又各具特色:
算法模型组:积极组队参加kaggle等比赛,原创手把手教系列文章;
调研分析组:通过专访等方式调研大数据的应用,探索数据产品之美;
系统平台组:追踪大数据&人工智能系统平台技术前沿,对话专家;
自然语言处理组:重于实践,积极参加比赛及策划各类文本分析项目;
制造业大数据组:秉工业强国之梦,产学研政结合,挖掘数据价值;
数据可视化组:将信息与艺术融合,探索数据之美,学用可视化讲故事;
网络爬虫组:爬取网络信息,配合其他各组开发创意项目。
点击文末“阅读原文”,报名数据派研究部志愿者,总有一组适合你~
转载须知
如需转载,请在开篇显著位置注明作者和出处(转自:数据派THUID:DatapiTHU),并在文章结尾放置数据派醒目二维码。有原创标识文章,请发送【文章名称-待授权公众号名称及ID】至联系邮箱,申请白名单授权并按要求编辑。
未经许可的转载以及改编者,我们将依法追究其法律责任。
数据派THU作为数据科学类公众号,背靠清华大学大数据研究中心,分享前沿数据科学与大数据技术创新研究动态、持续传播数据科学知识,努力建设数据人才聚集平台、打造中国大数据最强集团军。
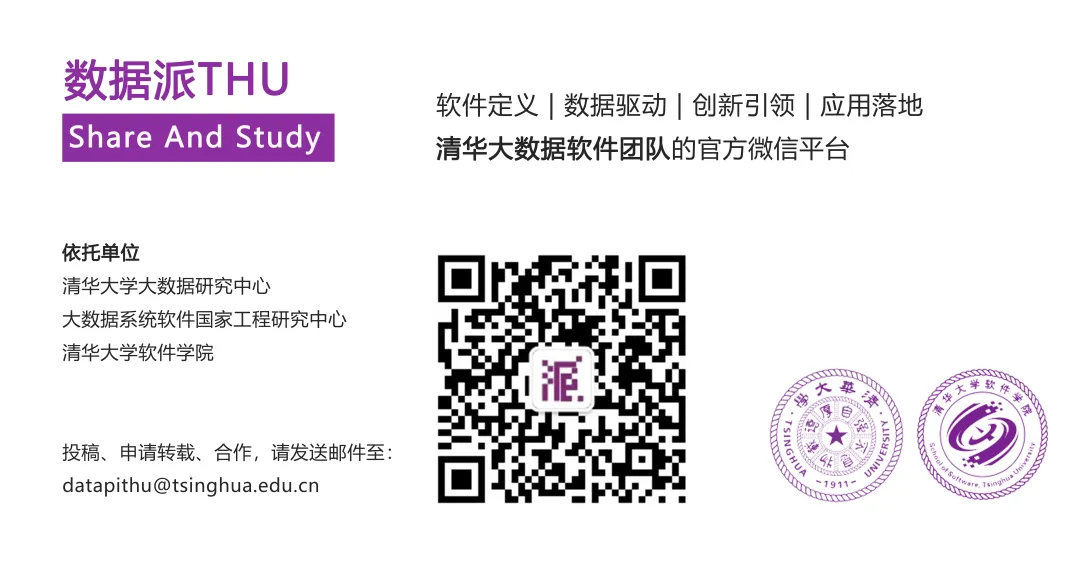
新浪微博:@数据派THU
微信视频号:数据派THU
今日头条:数据派THU