The Bayesian community should really start going to ICLR. They really
should have started going years ago. Some people actually have.
For too long we Bayesians have, quite arrogantly, dismissed deep
neural networks as unprincipled, dumb black boxes that lack elegance. We
said that highly over-parametrised models fitted via maximum likelihood
can't possibly work, they will overfit, won't generalise, etc. We
touted our Bayesian nonparametric models instead: Chinese restaurants,
Indian buffets, Gaussian processes. And, when things started looking
really dire for us Bayesians, we even formed an alliance with kernel
people, who used to be our mortal enemies just years before because they
like convex optimisation. Surely, nonparametric models like kernel
machines are a principled way to build models with effectively infinite
number of parameters. Any model with infinite parameters should be
strictly better than any large, but finite parametric model, right?
Well, we have been proven wrong.
But maybe not, actually. We Bayesians also have a not-so-secret
super-weapon: we can take algorithms that work well, reinterpret them as
approximations to some form of Bayesian inference, and voila, we can
claim credit for the success of an entire field of machine learning as a
special case of Bayesian machine learning. We are the BORG of machine
learning: eventually assimilate all other successful areas of machine
learning and make them perfect. Resistance is futile.
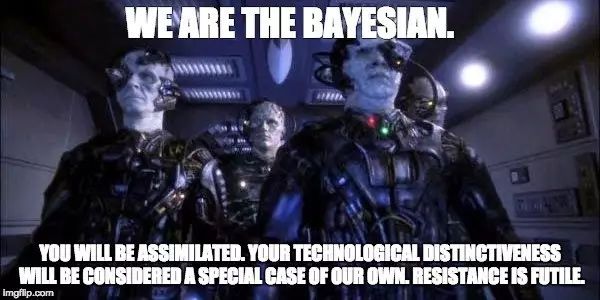
We did this before: L1 regularisation is just MAP estimation with
sparsity inducing priors, support vector machines are just the wrong way
to train Gaussian processes. David Duvenaud and I even snatched herding
from Max Welling and Alex Smola when we established herding is just Bayesian quadrature done slightly wrong.
But so far, we just couldn't find a way to claim credit for all of
deep learning. Some of us tried to come through the back-door with
Bayesian neural networks. It helps somewhat that Yann LeCun himself has
written a paper on the topic. Yarin managed to claim dropout is just variational inference done wrong.
But so far, Bayesian neural networks are just a complementary to
existing successes. We could not so far claim that deep networks trained
with stochastic gradient descent are Bayesian. Well, fellow Bayesian,
our wait may be over.
链接:
http://www.inference.vc/everything-that-works-works-because-its-bayesian-2/
原文链接:
http://weibo.com/1402400261/F4PWA7WYp?from=page_1005051402400261_profile&wvr=6&mod=weibotime&type=comment#_rnd1495804641101